Why you should adopt AI-powered planning tools sooner rather than later.
The role of AI in retail planning is getting clearer every day as the technology matures and leaders in the sector start to reap the benefits.
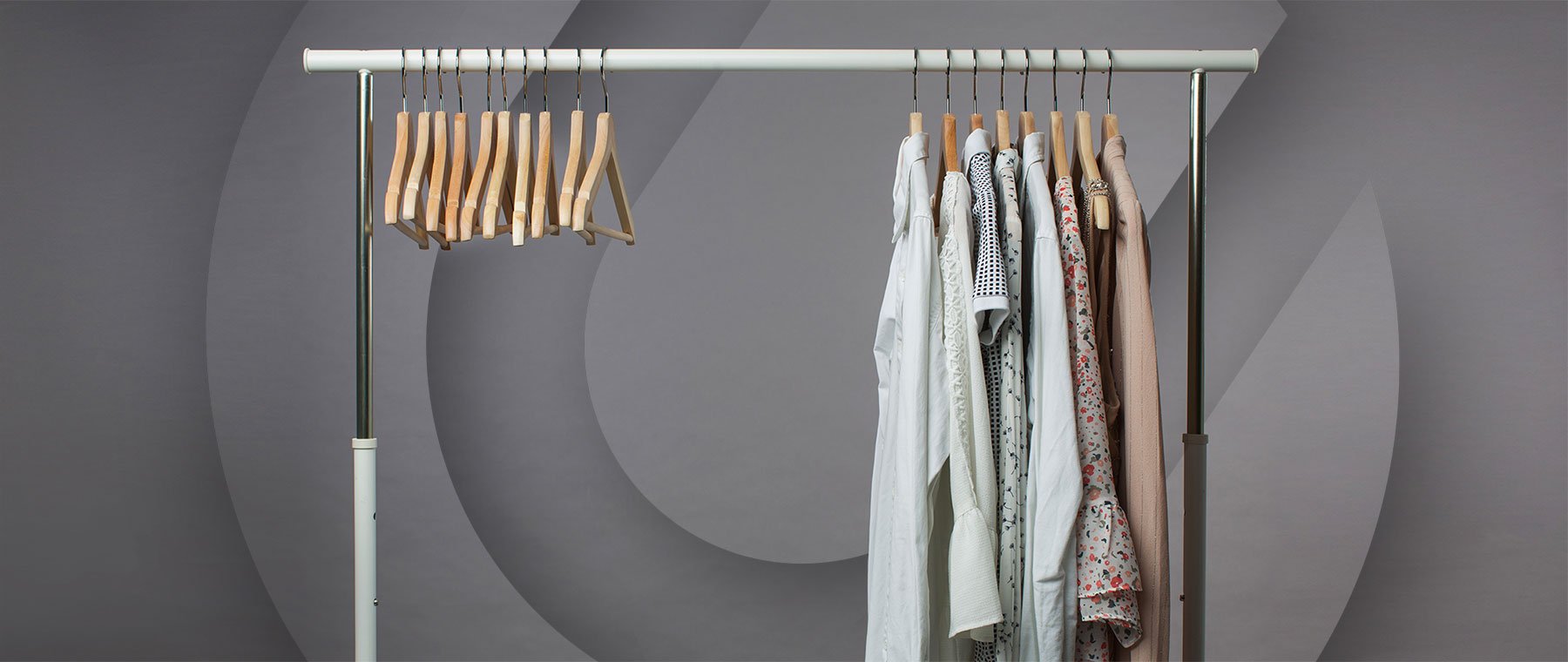
Implementing and embracing the right planning tool has the potential to impact a range of core business metrics that all ultimately relate to profitability. It’s reasonable to expect to see:
- Up to 40% improvement in stock turn, freeing up working capital and providing retailers with the flexibility and agility they need to focus on high-margin SKUs
- 35% improvement in forecast accuracy, driving confidence in future decisions on existing stock and imminent procurement decisions
- 85% accuracy on predecessor product, resulting in automated and accurate fulfilment, freeing up precious product management time
The financial bottom-line benefits are significant with the right stock being ordered, going to the right locations and customers in the right quantities, and accurate demand data flowing through the supply chain. In fact, in today’s super challenging market environment, AI is often the difference between red and black ink at the end of the financial year.
- What are the signs you’ve got a forecasting problem?
- Why is AI so much better than traditional methods and how will it impact planning processes?
- How do you get started implementing a solution like Quantiful?
What are the key indicators of poor planning accuracy?
Most businesses plan for short, medium, and long-term horizons. Over these three horizons, planning problems manifest themselves differently.
Short-term Replenishment Planning (or Inventory Optimisation) is where poor planning accuracy is most visible. Many companies look to optimise store-level inventory to mitigate lost sales and increase working capital efficiency, where it is often the most material.
Over the mid/longer range, poor planning accuracy is equally apparent, but to different operational teams -
“Everyone has a forecasting problem, because no one’s forecasts are ever 100% accurate; the question is whether your planning sessions have ceased to be strategic and turned into a tactical firefight because the forecast data that underpins them is of such poor quality.”
- Relationships with suppliers and manufacturers (yours or others) are strained due to poor upstream forecasting.
- No internal alignment within the business between sales, marketing, and finance leads to fraught planning meetings, leading to a lot of aged stock sitting in your DCs.
Of course, these things show up in the business financials, with lower sales, more working capital consumed, and declining profit performance.
Why is AI so much better than traditional forecasting than people?
For many organisations, demand planning is done by category managers in heavily customised Excel spreadsheets that consume historical sales data and output forecasts consumed by a central ERP. These models have varying degrees of sophistication but are all based on a statistical model that only forecasts future demand based on history.
Specialised software apps and planning modules in big ERPs have been around for a long time and are effective at varying levels. Nowadays, the algorithms that underpin them are usually AI-based. However, the problem is that these systems and processes are trying to plan for demand in an increasingly unpredictable world.
Over the last five years, supply chains have been massively disrupted. Customer buying behaviours may have changed significantly, and new competitors, often from low-cost source markets like China, have aggressively entered the market. As a result, the effectiveness and accuracy of their planning tools have declined, reducing trust in them and further deteriorating forecasting processes.
Demand planning in an uncertain time is a problem that AI is uniquely suited to solve. The computational power of AI and the correct training data deliver forecast accuracy outcomes at a scale that exceeds most manual systems. When considering AI, it is valuable to understand what AI does differently from traditional statistical forecasting models, which explains why their outputs are so much better.
The critical difference is multi-time series forecasting and using external data to augment historical data. They also take a ‘whole-of-portfolio’ approach to understanding the interdependencies inside your product set. For example, Quantiful will automatically correct/change the demand profile for related products if a price change or promotion occurs. As a result, Quantiful’s demand plans at a portfolio level are far more accurate than alternative systems that lack the processing power to execute scenarios across a portfolio.
How do you get started?
It’s much easier to get AI-powered forecasts than most people think. Quantiful, for example, has been designed to exchange information quickly and easily with all types of IT and ERP systems without extensive integration. It uses SFTP-secured batch files to move data around, and you can be up and running in 60 days or less.
The steps below to get started should be well within the capabilities of all retailers, even those with highly customised IT systems.
Step 1 - The first step is to determine where to start - identifying an SKU or a product line to plan for. With a clear objective and performance metrics, any pilot or POC will be more than sufficient to validate an entire business case.Step 2 - the next step is to gather enough historical data to train the AI. AI is beautiful because it can complete any missing data, transform any disorganised data, and back-test your history so you can start with the best possible model for your products.
- Minimum 2-3 years of sales history
- Inventory data
- Master product data (specifications, colours etc - the richer the better)
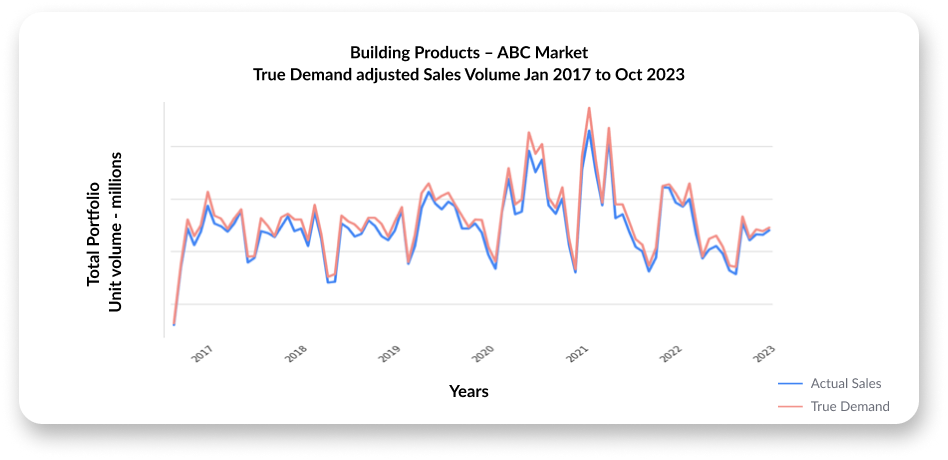
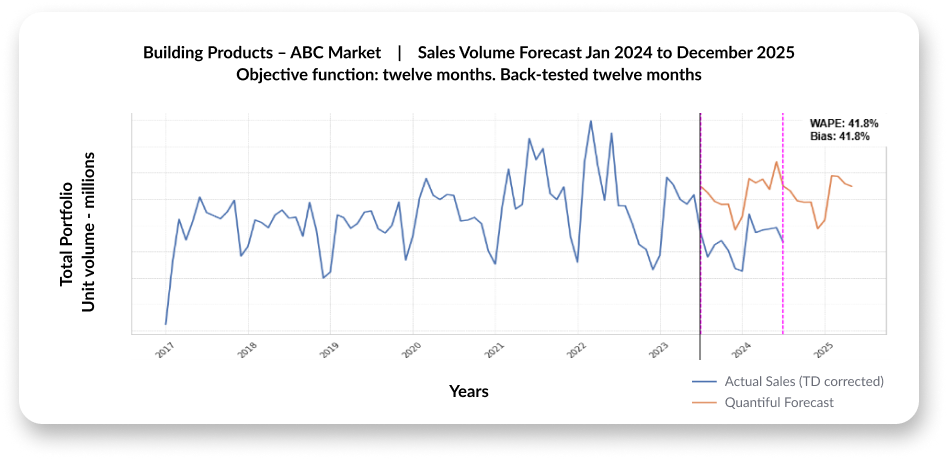
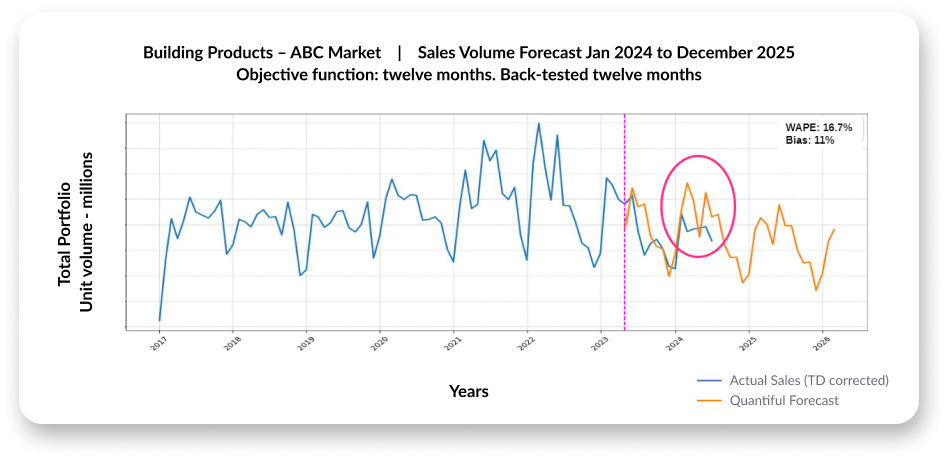
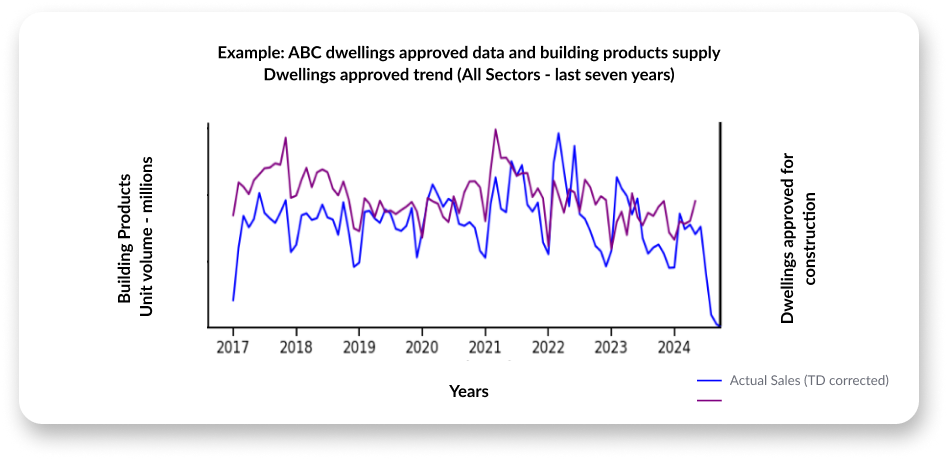
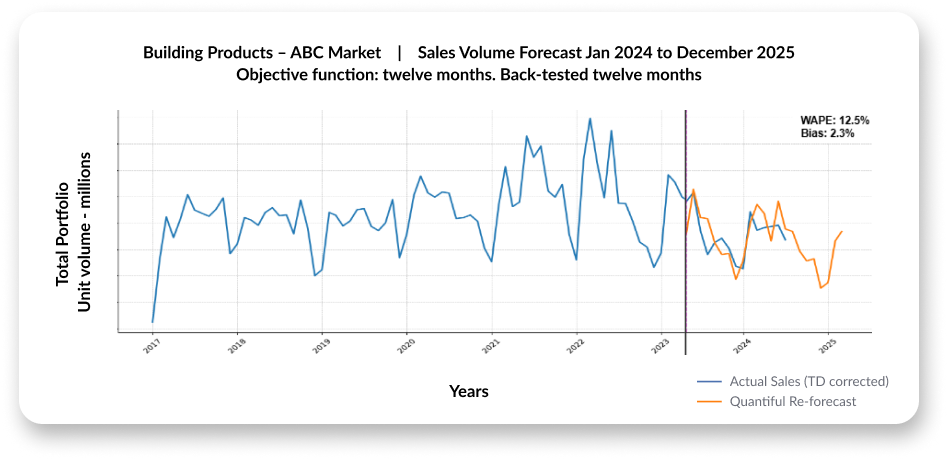
Once all parties are happy with the accuracy of the forecast we can begin supplying them on a daily, weekly or monthly basis - whatever works for you.
Conclusions
It is critical to build your S&OP processes on an accurate data foundation. AI has proven to be the right technology for Demand Planning, and organisations that embrace it thrive.
However, the belief that it’s time-consuming, lengthy and complex to implement is incorrect. In our experience, we can deliver accurate forecasts to ANZ customers in 60 days or less.