Has truly optimised replenishment been possible until now?
The word “Optimisation” has been buzzing around boardrooms for many years now. Choosing to constantly measure performance, learn from findings and refine accordingly is a wise move and this process has been adopted by most, if not all, high-performing businesses. These organisations are likely to not just survive, but thrive in challenging times.
But the question is this - are these processes (usually based on historical data generated by their business operations) truly “optimised”? Or is AI, ML and Big Data bringing true optimisation to the table, by allowing organisations to react to market anomalies before they even happen?
Old school optimisation
It’s widely known that an optimised system can help move businesses away from more blunt force instruments of planning like rigid minimum / maximum stock levels. Regularly testing and adjusting replenishment processes gives Demand Planners the ability to create or change supply orders, executing these changes automatically or as a one-off.
The benefits of doing this include:
- Minimised lost sales
- Reduced risk of obsolescence/spoilage of goods due to inventory excess
- Reduction in waste levels
- Increased planning efficiency and visibility of stock requirements
- Streamlined inventory and related costs
- Optimal stock movement and storage throughout the supply chain
But the reality is that a system that’s built on retrospective information can only achieve so much.
Optimisation that PREDICTS as well as measures / adjusts
Let’s consider the two main elements of optimised replenishment - CPFR (Collaborative Planning, Forecasting and Replenishment) and Time Phased Planning - and consider how AI and ML can enhance these processes.
1: CPFR
At its core, Collaborative planning, forecasting and replenishment means that knowledge and information is shared throughout the supply chain to eliminate some of the uncertainty associated with supply and demand. Initially developed for Walmart, this process boosts operational efficiency by using demand forecasting based on data points captured throughout the supply chain and even from the customer, to ensure stock levels meet (but don’t exceed) demand.
CPFR is a process of continuous improvement and will only be effective if it’s systematically implemented. A truly optimised supply chain can help you deliver your goods accurately, in line with customer demand and as cost-effectively as possible.
CPFR is a process of continuous improvement and will only be effective if it’s systematically implemented
This process - alongside Artificial Intelligence, Machine Learning and Big Data - is a powerful combination. While CPFR certainly predicts consumer behaviour based on the knowledge shared across a supply chain, incorporating wider consumer data allows businesses to forecast demand even more accurately. With all the right elements of an optimised replenishment system in place, they can meaningfully impact performance by increasing stock turn and maximising ROI across their organisation.
2: Time phased planning
This element of optimised replenishment is most relevant to retailers who manufacture products or goods, where raw materials are required to fulfil production requirements and in turn, customer demand. It is a Material Requirement Planning procedure that works to a predefined time frame. So if a vendor always delivers a material on a particular day of the week, you would plan this material according to its delivery cycle.
It works by assigning materials with an MPR date in the planning file, which re-sets after each planning run. By time-phasing material requirements, a good system can express future demand, supply and inventories by time period. The system will also delay the release of orders until they are needed, reducing storage costs and saving processing time.
Again, the more information you can feed into time phased planning, the better. A truly optimised system will draw on as much data as possible, providing accurate historical information as well as predictions that provide visibility of indicators. Big Data is a game-changer here. Add ML, AI and clever technology that allows people to override automation (because there are some business variables that can only be known by humans) and you have a process that can take replenishment optimisation to new levels.
QU - a replenishment planning tool for the times
QU is an AI powered SaaS demand forecasting tool that has been developed to measure, analyse and predict product performance in real time. Using the latest AI technology along with proprietary ML algorithms and innovative technology design, QU draws on millions of data points from hundreds of sources. It presents powerful insights in an easily-digestible and customisable dashboard that not only predicts buying behaviour, but adjusts forecasts of future sales right down to individual product level (SKU).
QU is a tool that is designed to empower human beings, giving them the insight and control they need to do better business.
So if your business is ready to start freeing up resource and firing up profitability…
Share this
You May Also Like
These Related Stories
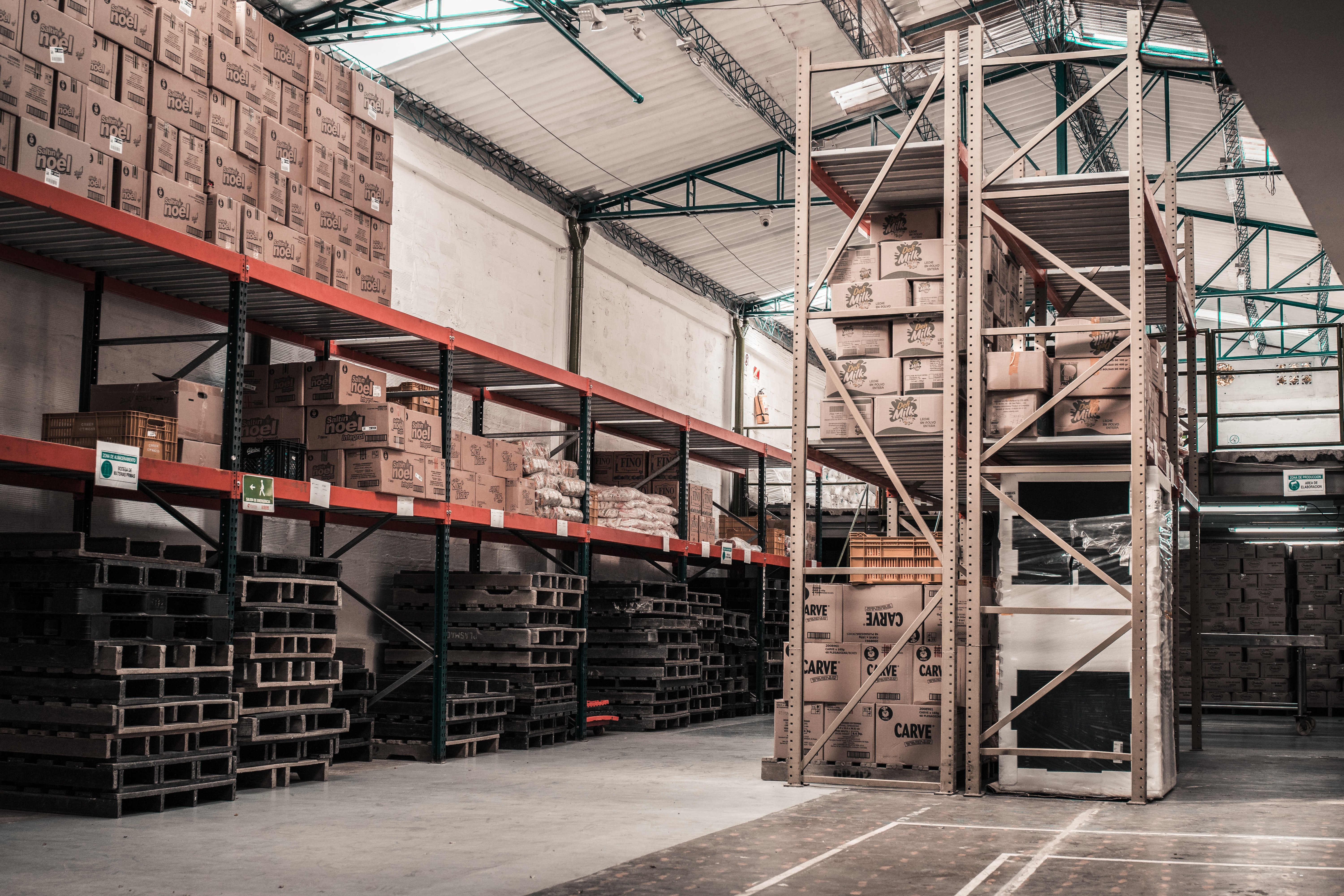
Demand forecasting for new products
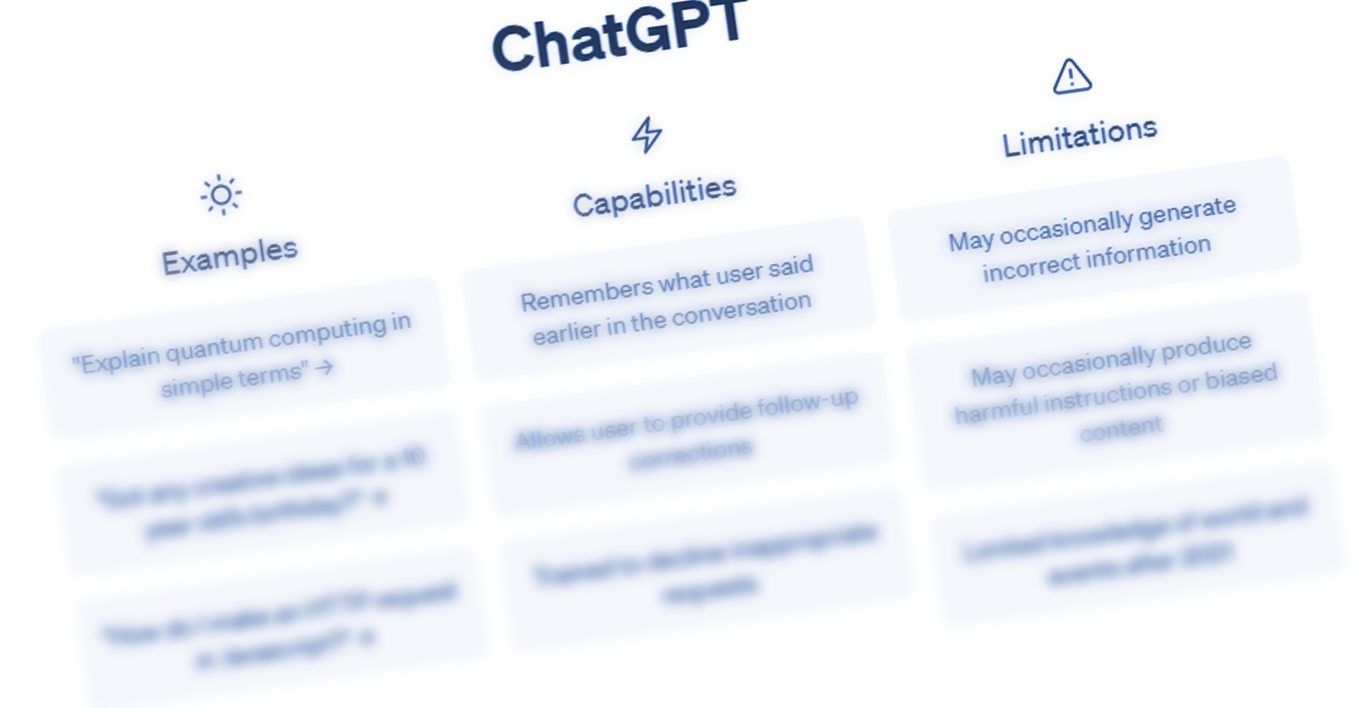
Will Generative AI tools like Chat GPT/GPT-4 replace Demand Forecasting tools?
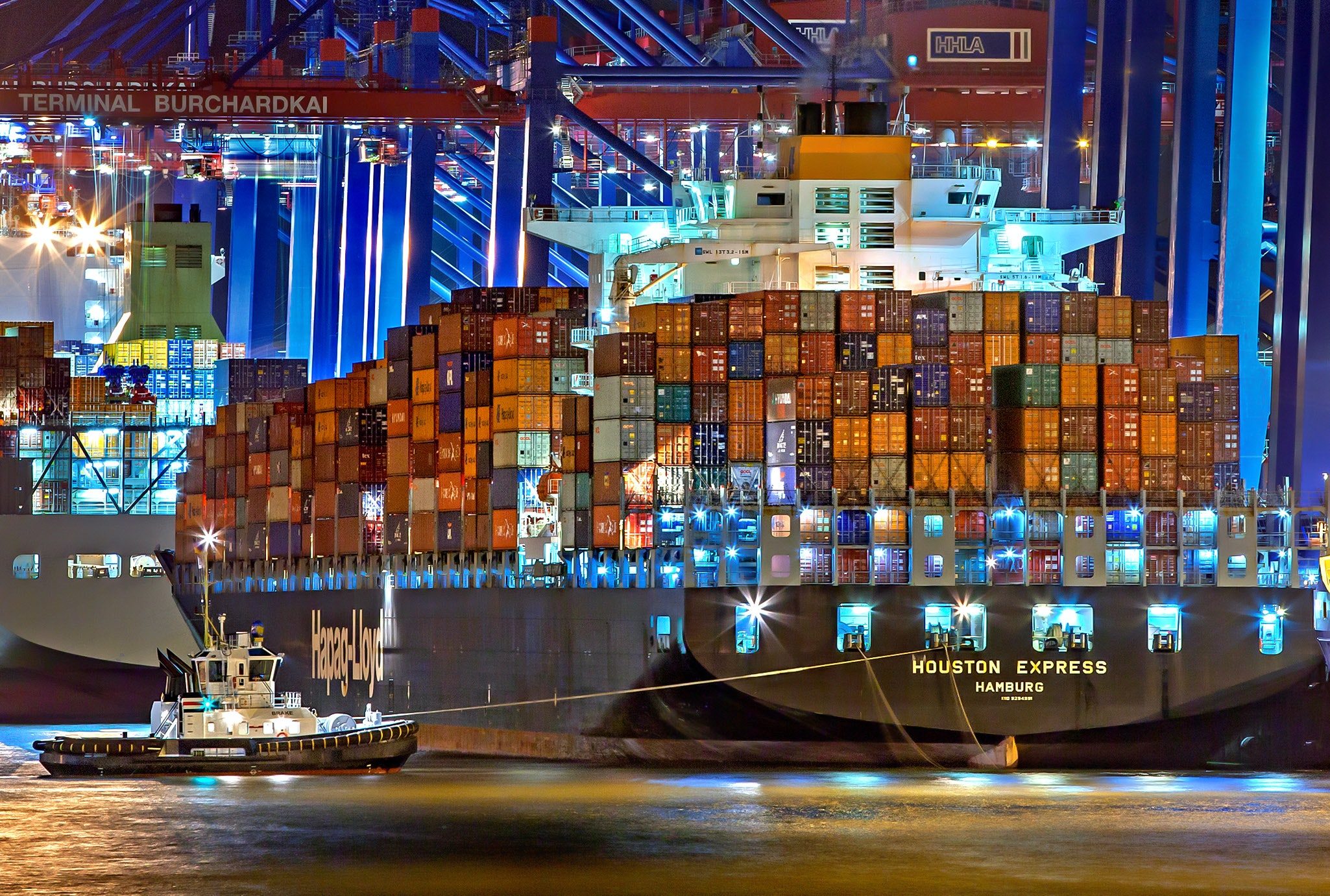