Will Generative AI tools like Chat GPT/GPT-4 replace Demand Forecasting tools?
Given what Quantiful is famous for, this question has come up more than once around our office recently as thousands of new use cases deploying this tech come to light. Forecasting demand has always been a critical aspect of business planning, and in the last few years, artificial intelligence (AI) has revolutionized forecasting.
Tools like Quantiful’s QU have been at the leading edge of this revolution and are used by our customers to predict market demand with remarkable accuracy. However, the obvious question is, can Generative AI tools like Chat GPT/GPT-4 replace QU, or does it, in fact, further strengthen it by adding to its capability?
So we were fascinated by a recent article in a Supply Chain Movement newsletter quoting AB InBev’s use of Chat GPT/GPT-4 to improve their forecasting.
It prompted us to explore the topic further.
In this blog post, we explore the capabilities and limitations of Generative AI tools like Chat GPT/GPT-4 for demand forecasting and consider three options. They are a replacement; they can play no role, or the combination of the two is most potent.
So let's look at the top four ways generative AI can help a Forecaster forecast better. We thought the best way to do this was to ask Chat GPT/GPT-4. Here is what it told us about its forecasting capabilities:
1. Uncover Hidden Patterns: One of the most significant advantages of using AI like Chat GPT/GPT-4 in demand forecasting is its ability to recognize patterns in large datasets that human analysts might overlook. This makes it extremely valuable for analyzing historical sales data and identifying trends that predict future demand.
2. Natural Language Processing: GPT-4 is trained on a diverse range of internet text and can be used to analyze qualitative, unstructured data, such as customer reviews and feedback, to identify shifts in consumer sentiment that could impact future demand.
3. Easy Integration: Chat GPT and similar tools with API access into their functionality can be integrated into third-party software tools relatively easily.
4. Providing Contextual Understanding: A significant advantage of Generative AI tools is their ability to understand the 'why' behind specific demand patterns. This can help businesses forecast the demand and understand it more deeply.
The next step was researching Chat GPT/GPT-4 limitations when forecasting demand. Here we used several sources, and while opinions varied, there are probably at least two that were cited most often as significant limitations of generative AI as a standalone capability for demand forecasting:
1/ Its inability to Interpret Raw Data: Data like graphs, images, and, most importantly, raw numerical data directly cannot be processed by Chat GPT/GPT-4 or similar tools. This limitation restricts its utility for demand forecasting as a starting point for this modeling is an analysis of raw transactional data like sales and inventory data.
2/ No Real-Time Learning: Generative AI tools don’t learn from their predictions or adapt to new events or trends in real time. The best-specialized forecasting tools use large external data sets for this purpose and can forecast multiple time series to improve accuracy. So except for the limited amount of data that can be included in a prompt, Generative AI tools cannot do this. Their training is static, based on the data it was initially trained on, and it cannot update or learn from new data or events.
So, in summary, where does this leave us:
In fact, at option three, the combination of Generative tools and a Specialised Forecasting tool is even more powerful than the two alone.
For example, the AI models in Specialised Forecasting tools are explicitly trained for Forecasting on structured data, and the good ones use multiple external data sets, all of which have signals in them, which will improve forecast accuracy. These data sets are often tailored to specific industry verticals.
However, the quality of data needs to be assessed and assured; garbage in and out still applies, and Planners need to have confidence in the data going in.
This is where Generative AI can be a powerful adjunct to Specialised forecasting tools; by using it to analyze the external data fed into them, more signals can be identified, which can then be extracted and fed into the models. In short, Generative AI can make the unstructured data structured and consumable by the scalable forecasting AI behind tools like QU.
Also, once a forecast has been generated, generative AI can then be used to summarise and provide clear text-based insight into the basis of the predictions in the forecast.
QU from Quantiful
QU is a specialized demand forecasting tool designed to onboard and scale rapidly in Enterprise Retail, Manufacturing, and Media. It uses customer sales and inventory data and multiple sets of custom external data such as search, social, and a host of others, amplified by Generative AI, to create highly accurate demand forecasts.
Share this
You May Also Like
These Related Stories
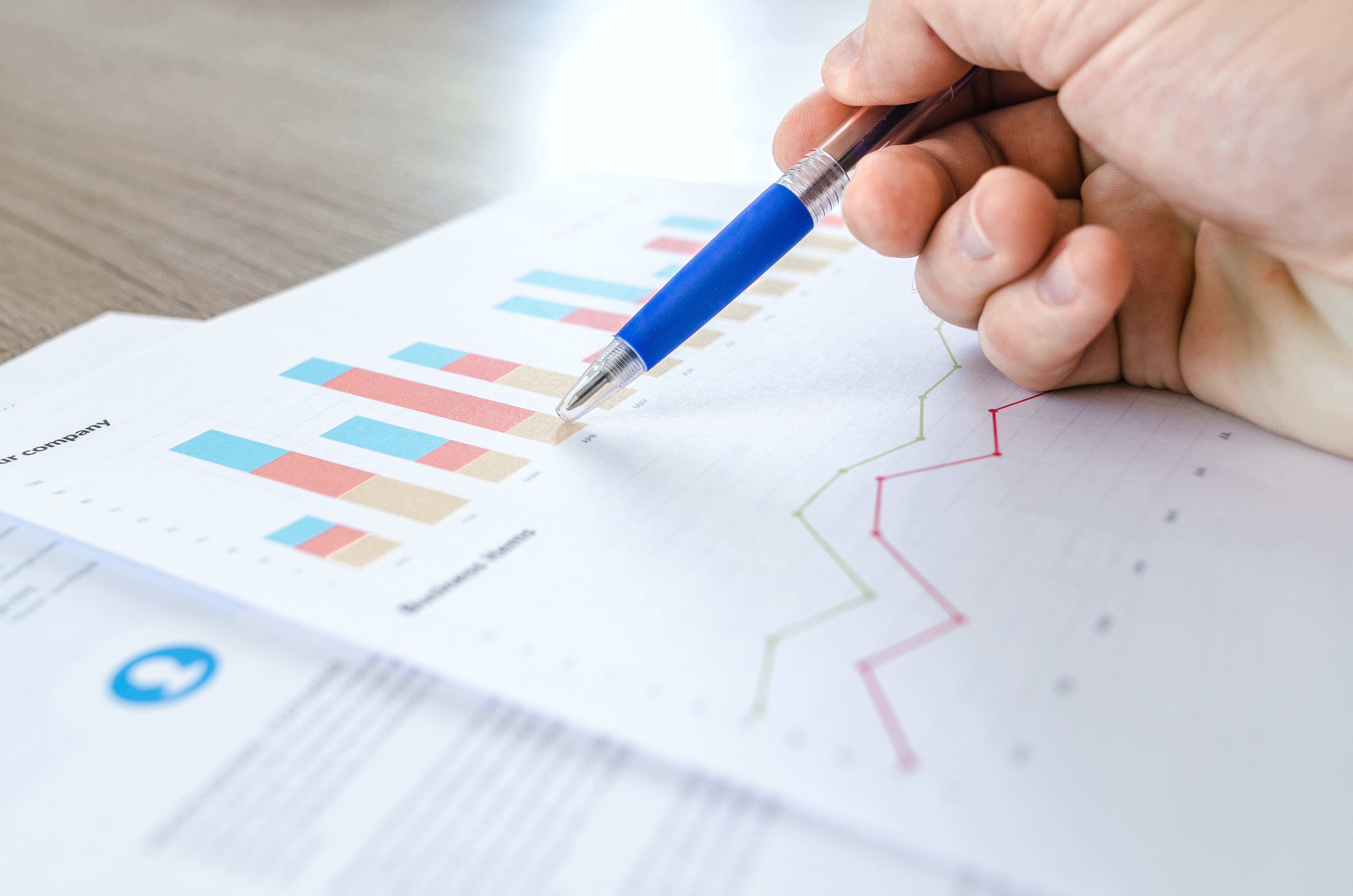
Top 10 Short-Term Replenishment Forecasting Challenges Retailers Face
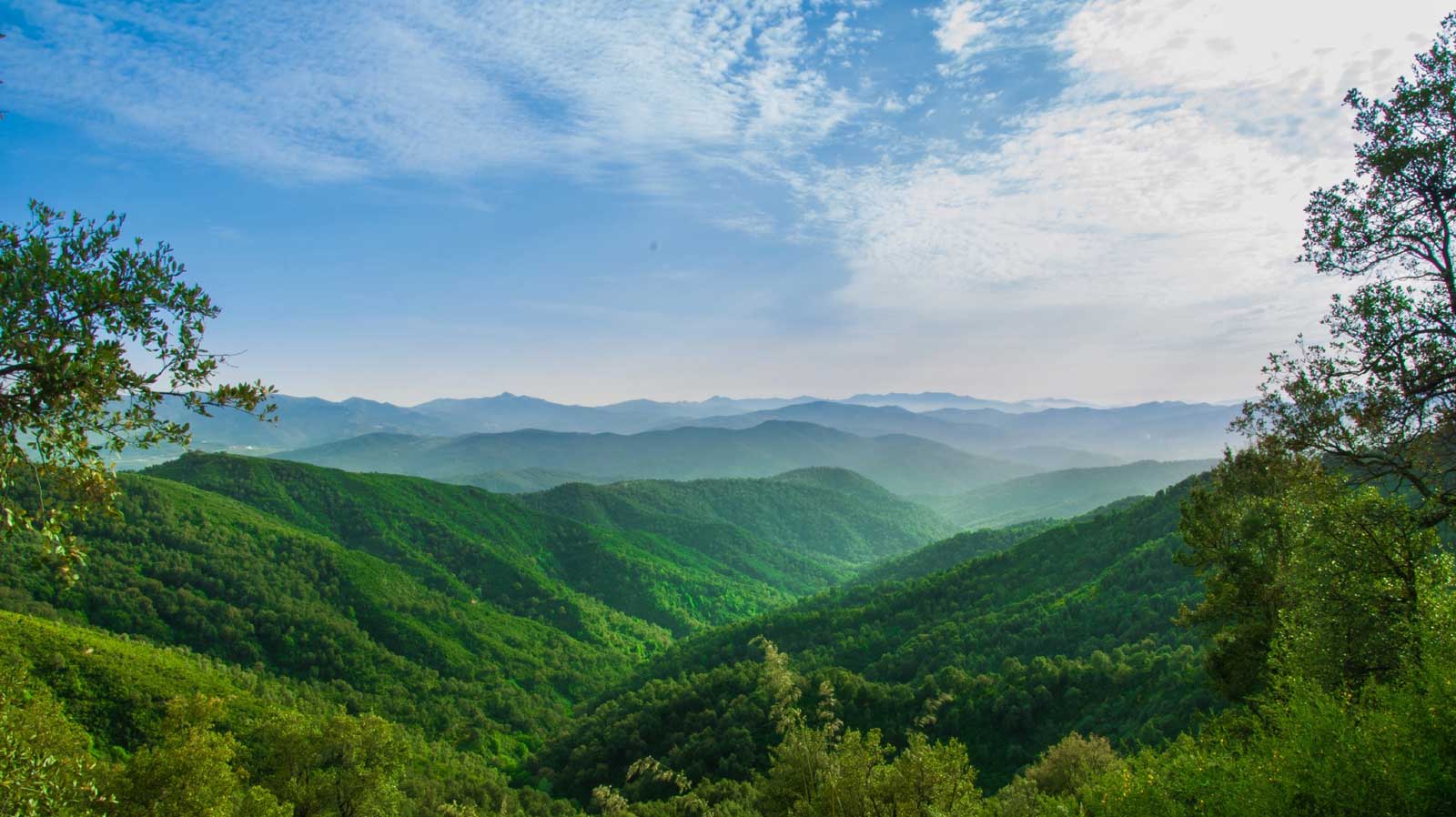
How QU Takes the Guesswork out of Pre-launch Planning
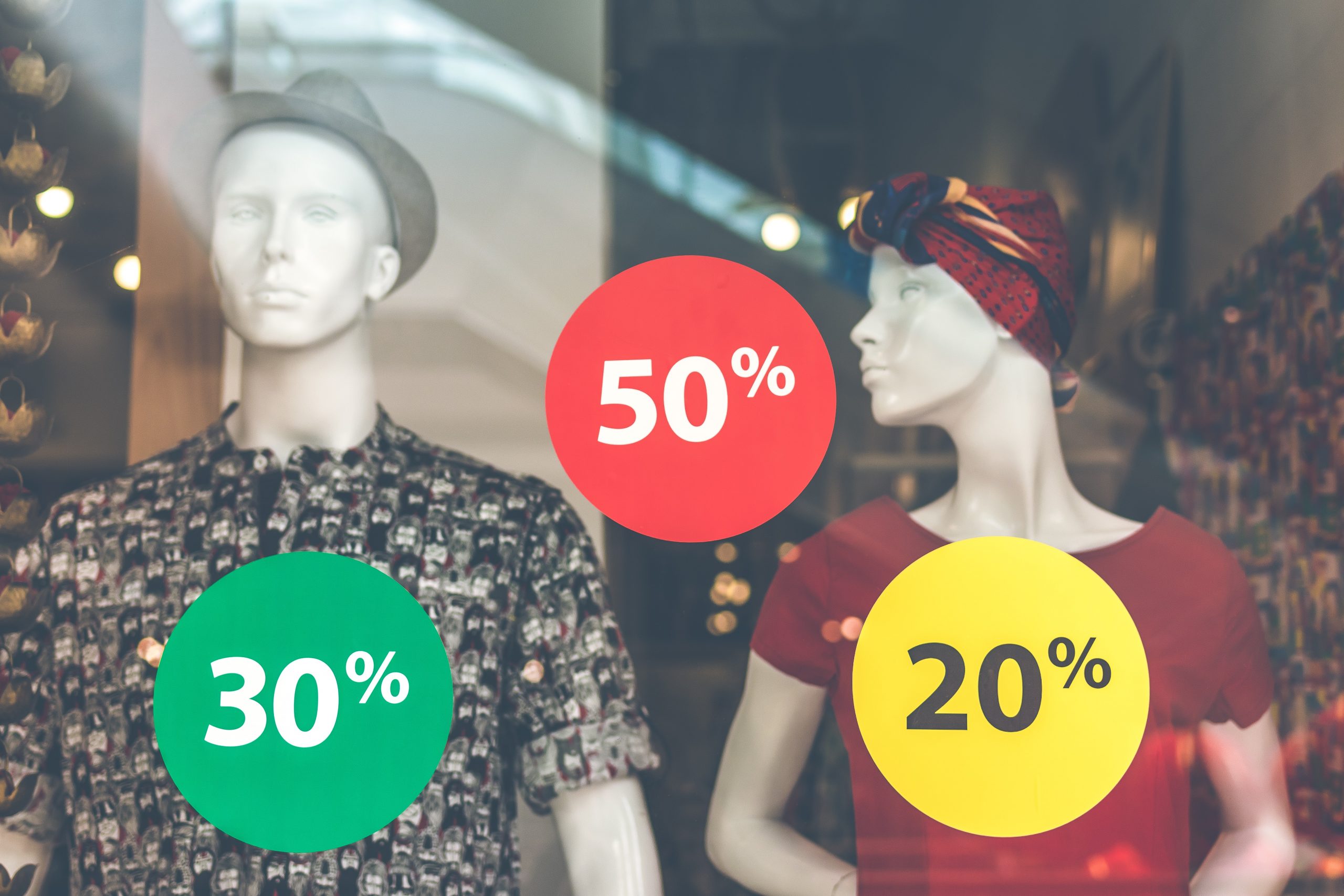