Enhance your demand pattern analysis
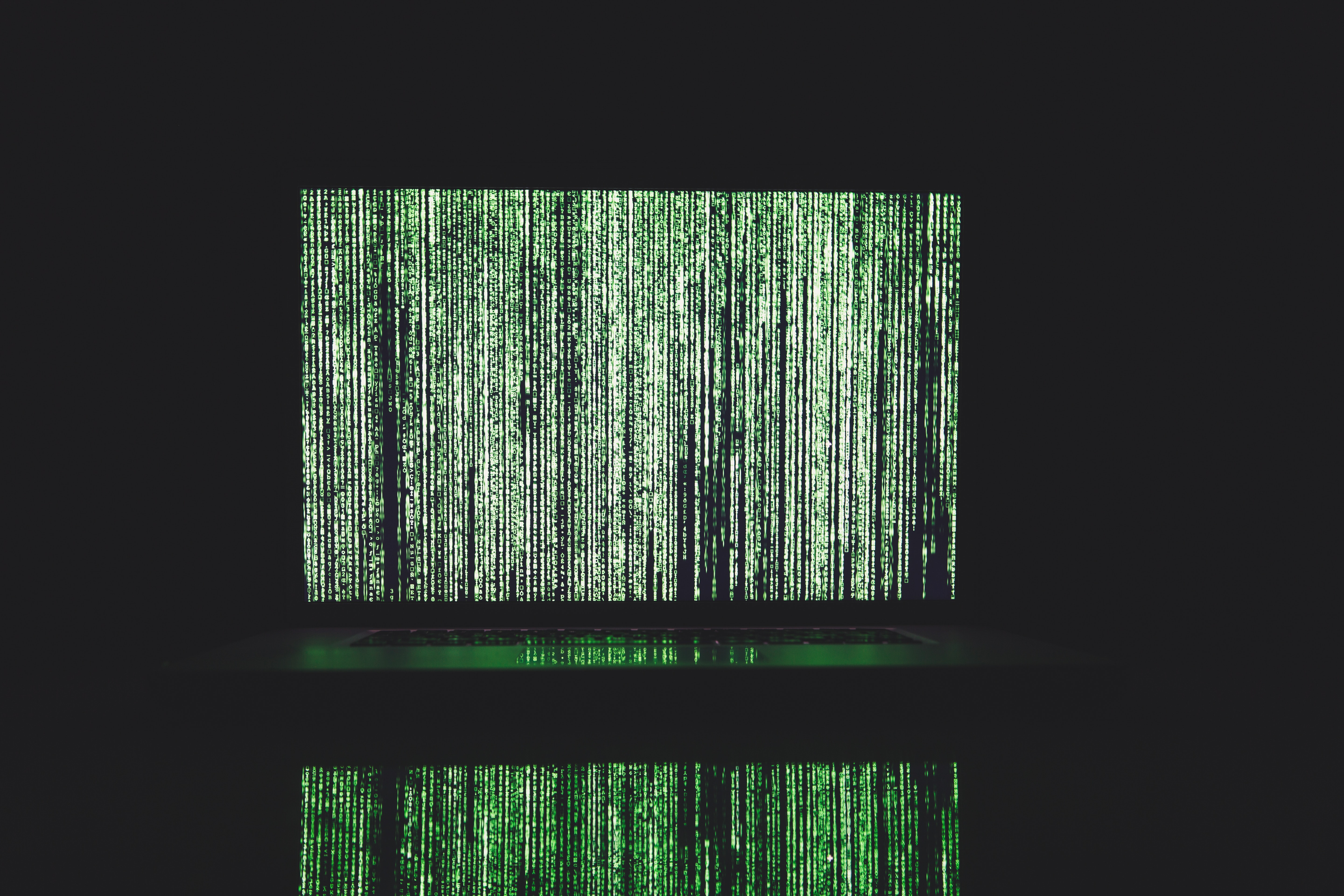
Enhance your demand pattern analysis
27 July 2022
2
min read
Integrate demand and supply planning
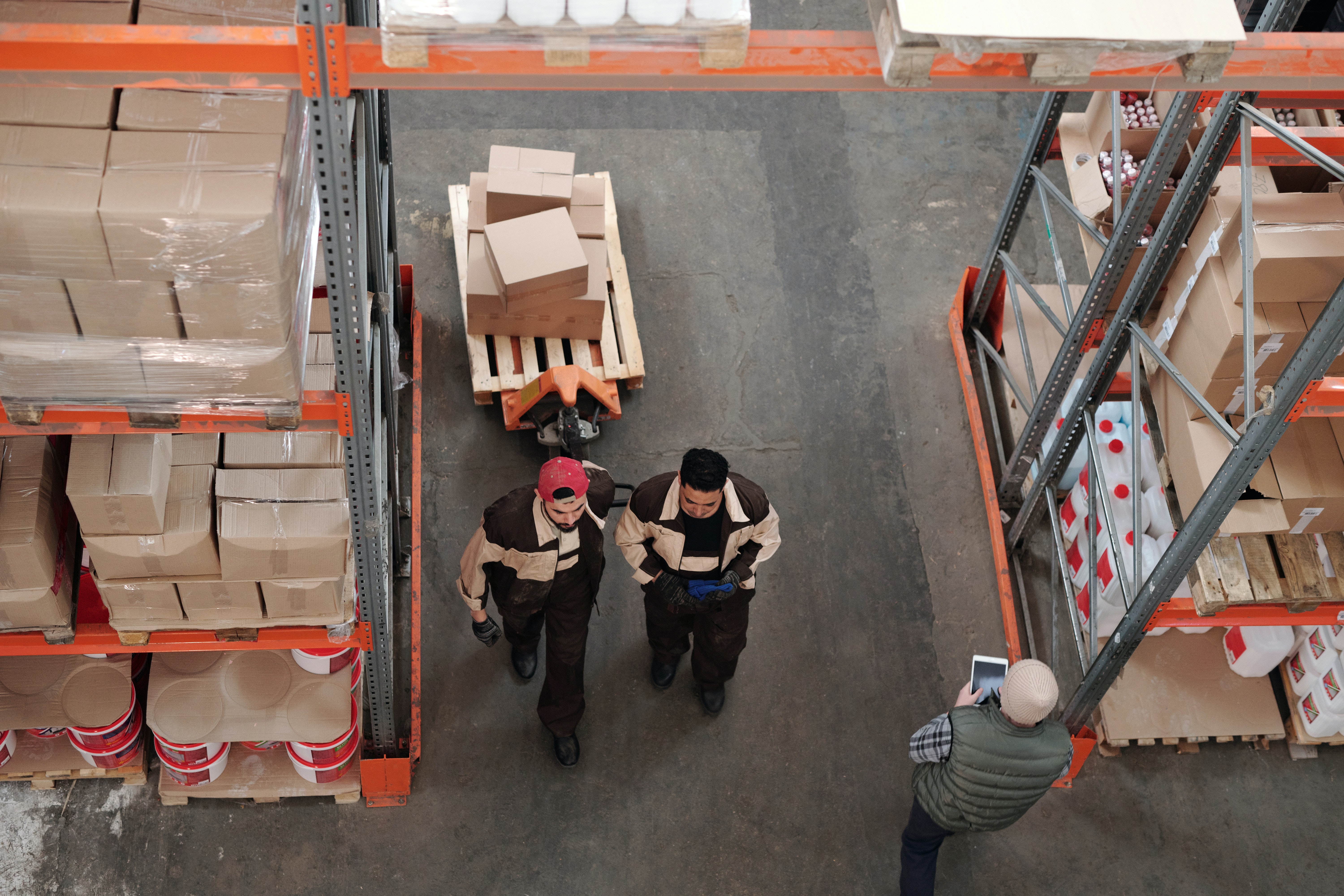
Integrate demand and supply planning
27 July 2022
2
min read
Compare SCM Tools
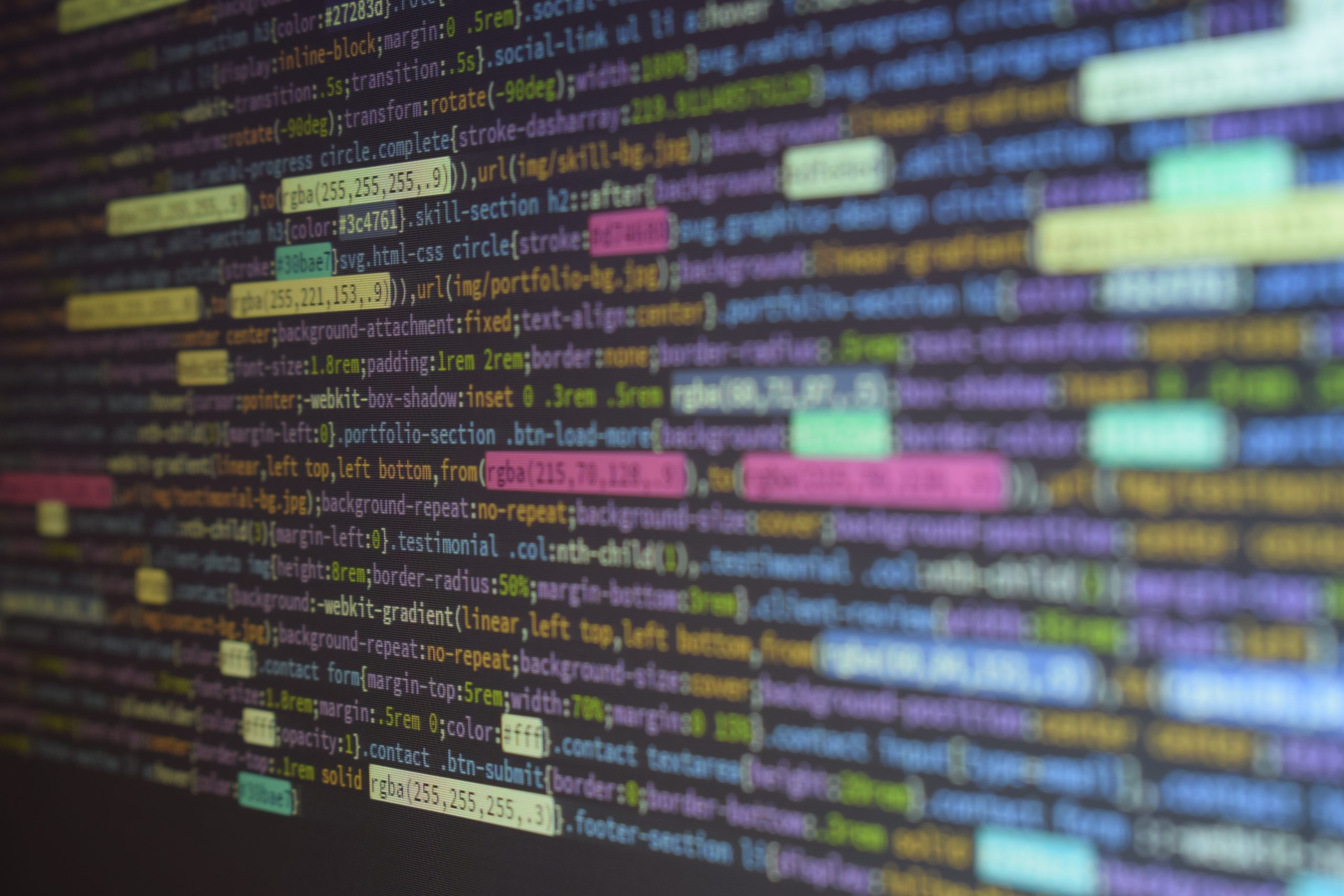
Compare SCM Tools
21 July 2022
1
min read
Demand forecasting for new products
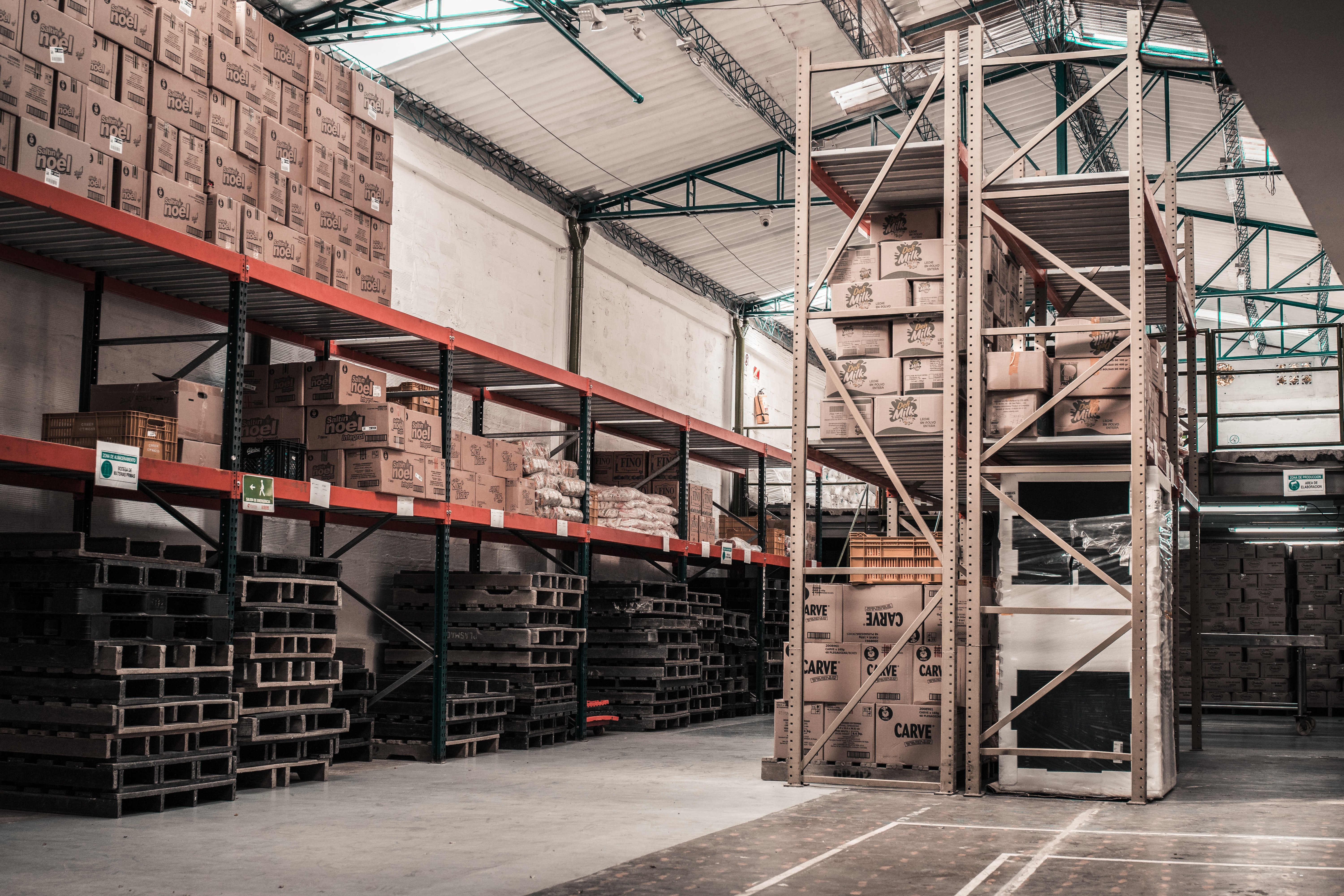
Demand forecasting for new products
19 July 2022
2
min read
Level up your Demand Planning
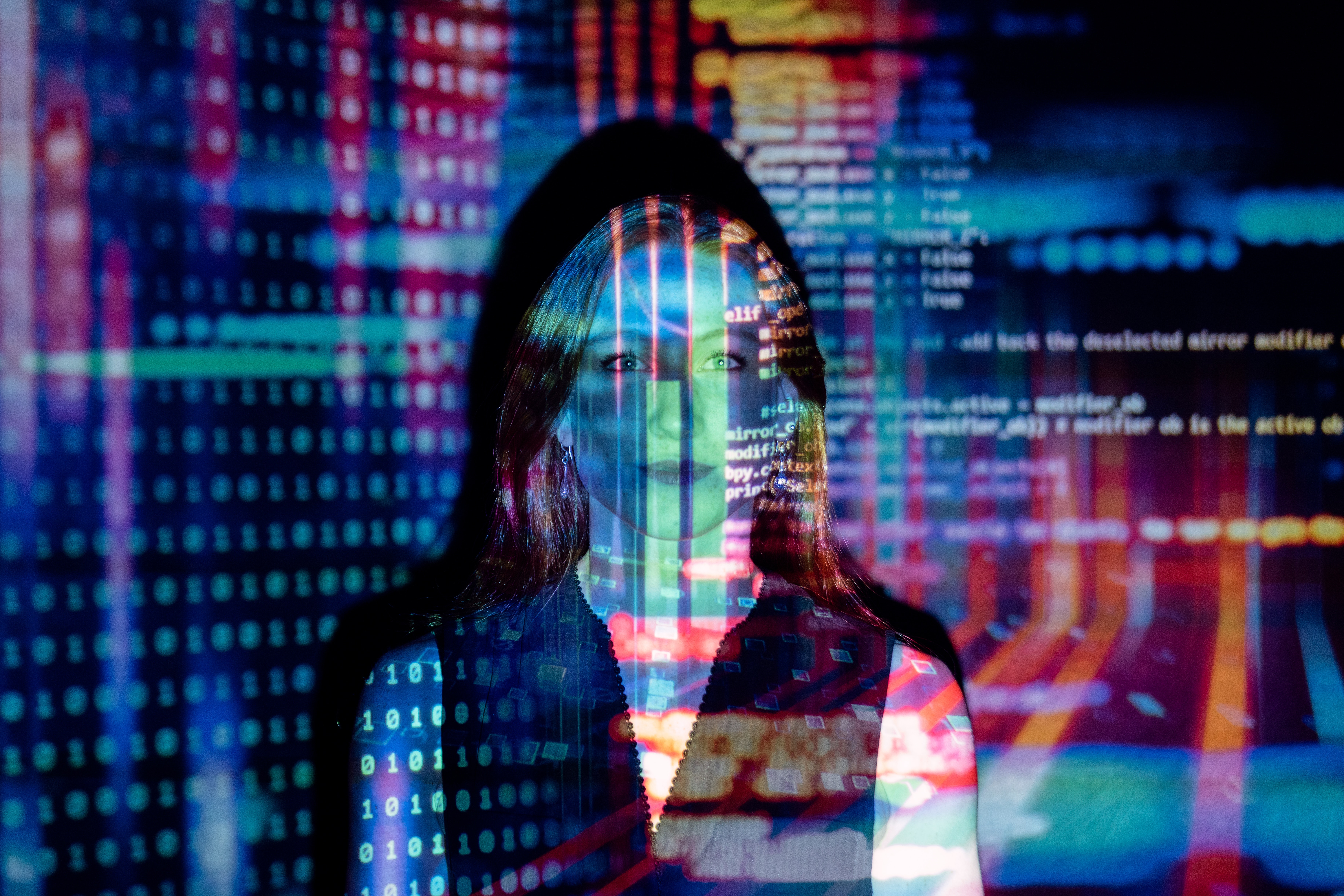
Level up your Demand Planning
19 July 2022
2
min read
Revolutionise your demand forecasting
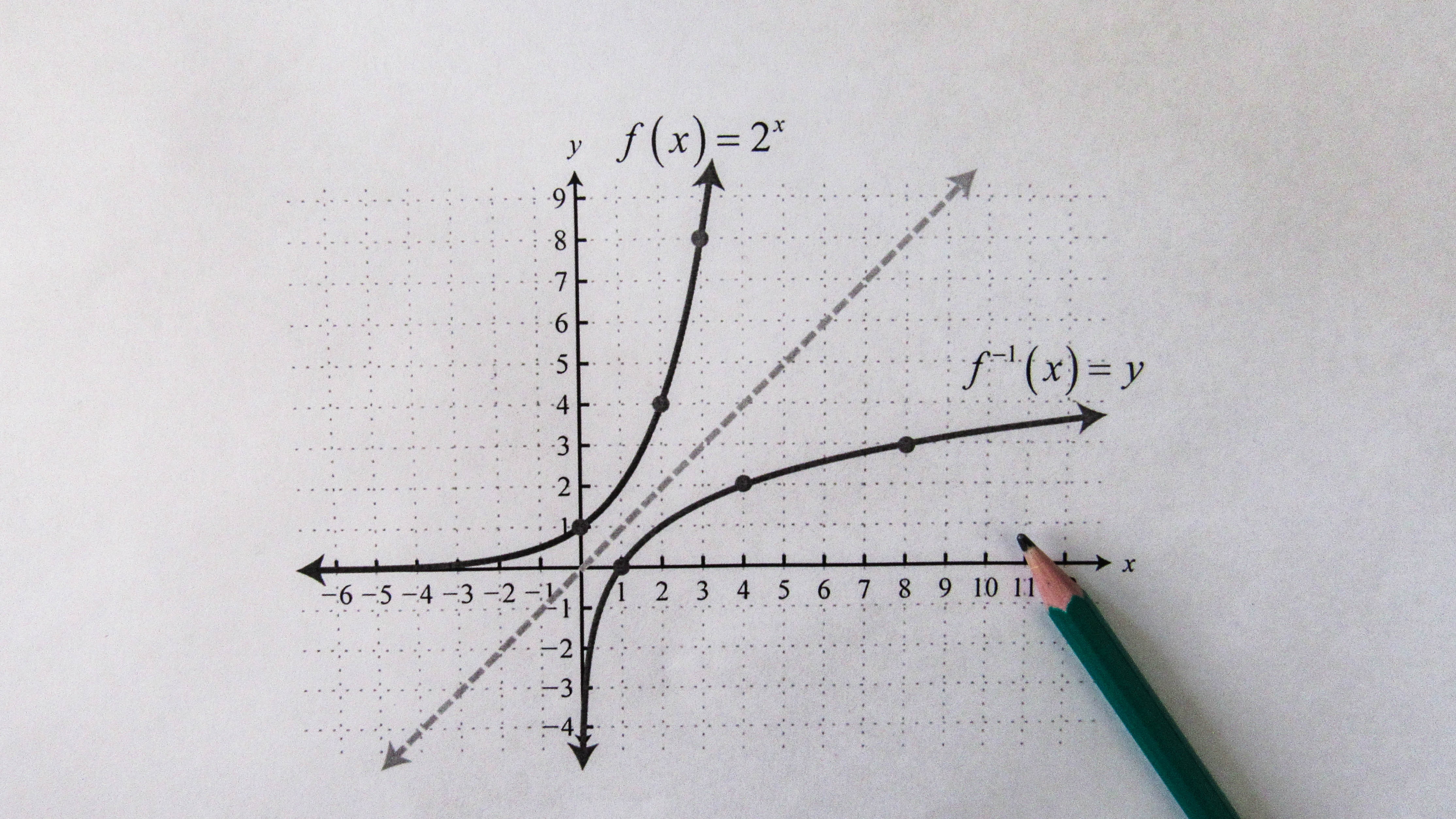
Revolutionise your demand forecasting
19 July 2022
2
min read
Demand forecasting by store
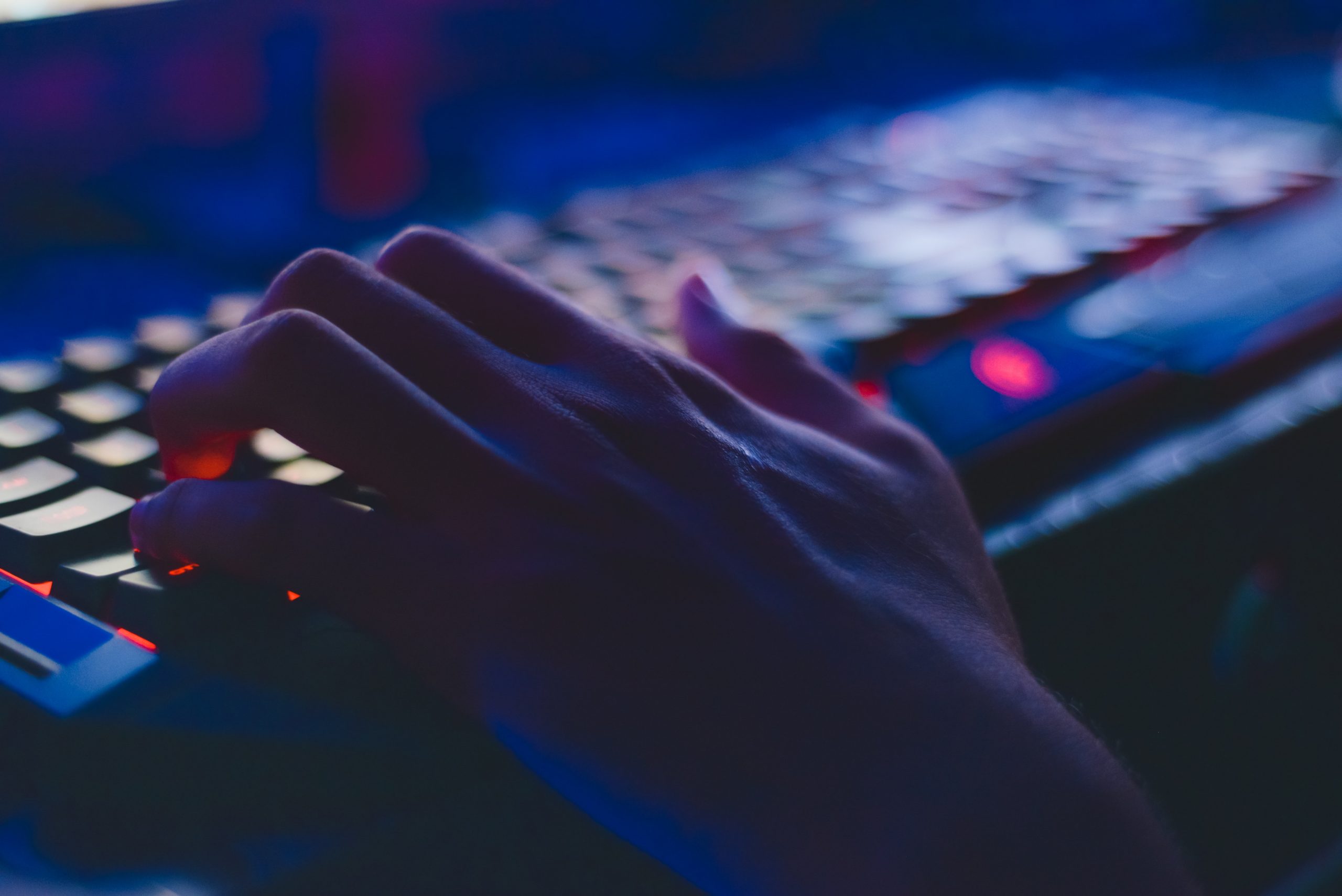
Demand forecasting by store
19 July 2022
2
min read
Move from demand forecasting to demand sensing
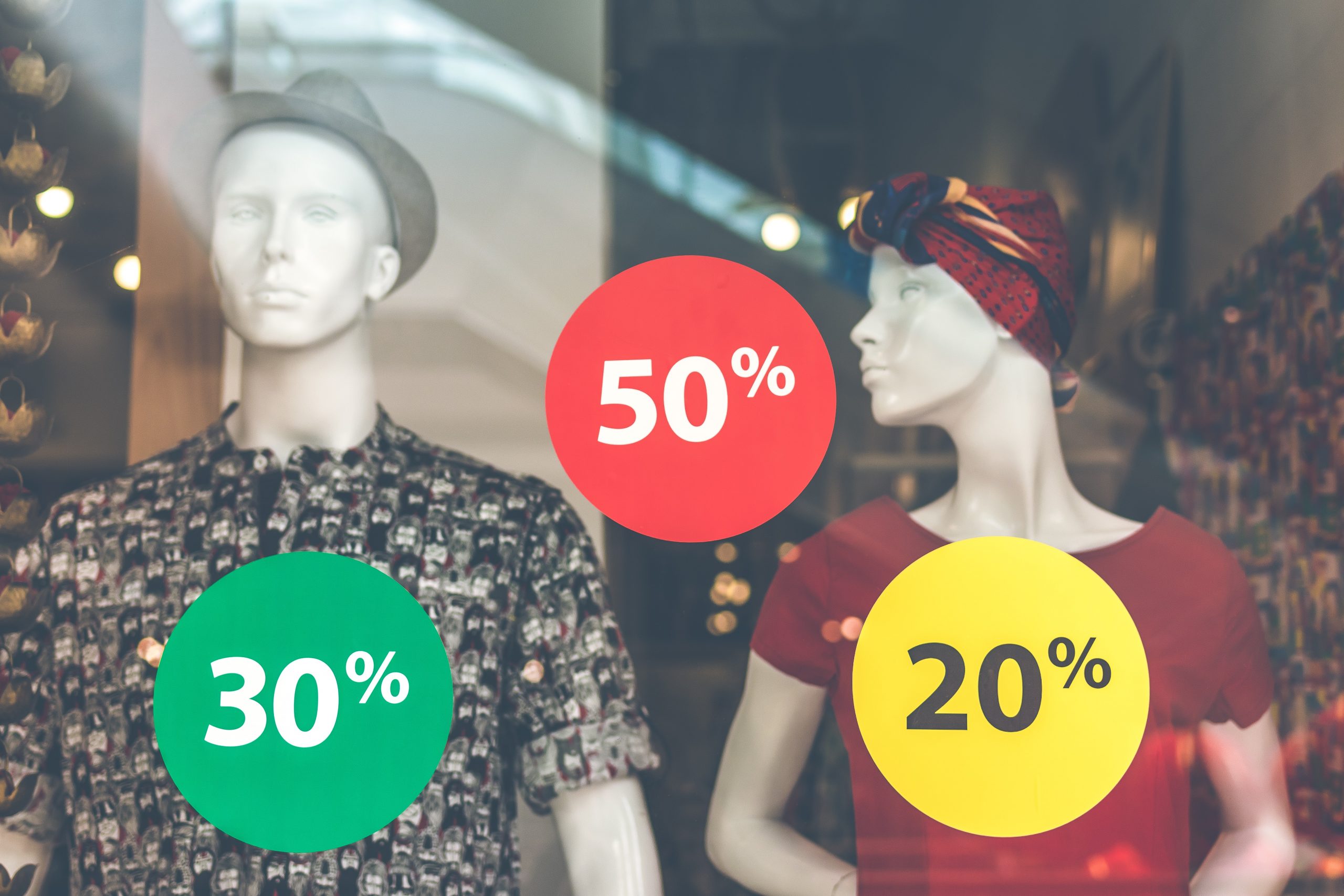
Move from demand forecasting to demand sensing
19 July 2022
2
min read
Optimise your SKU productivity
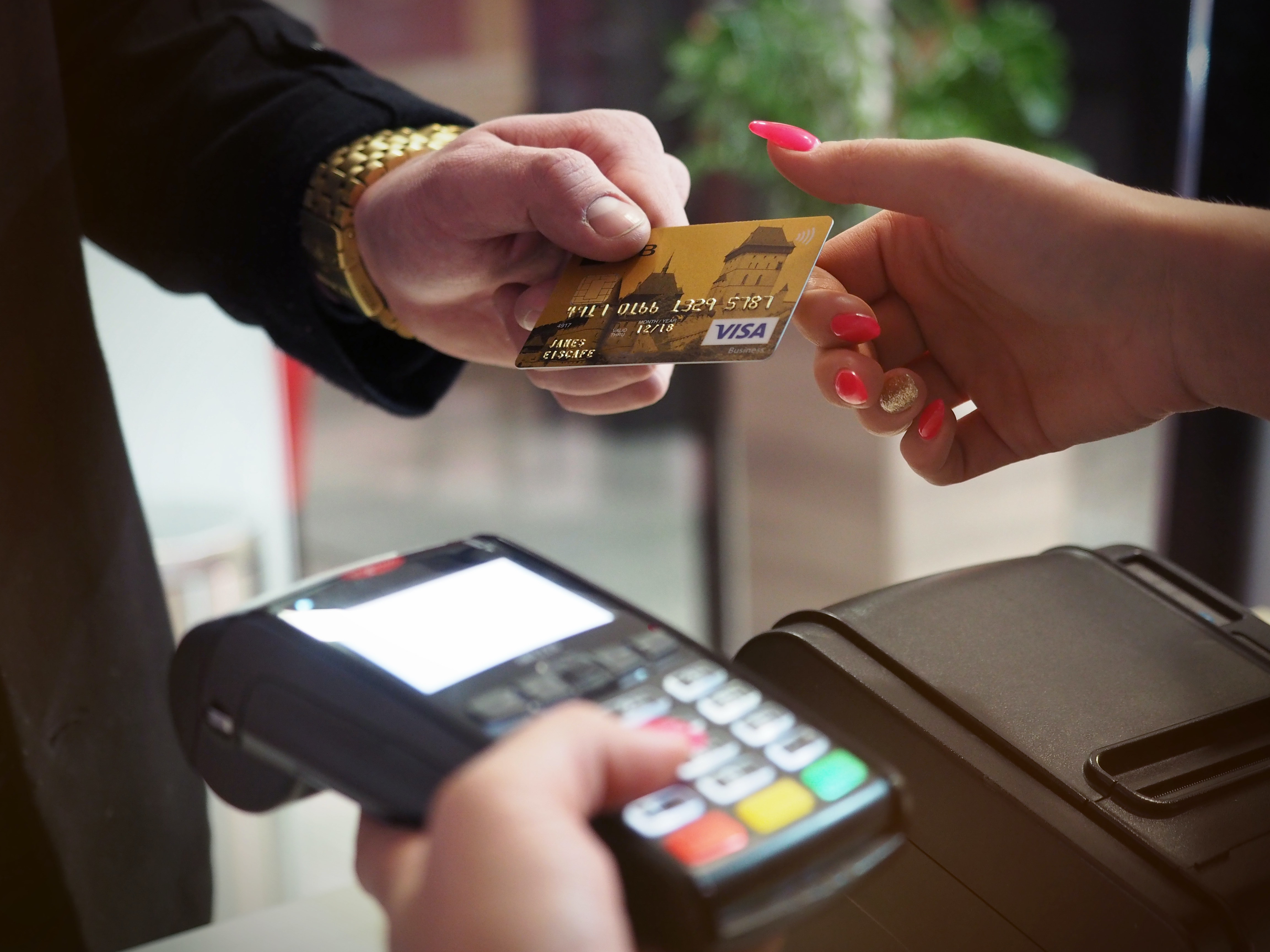
Optimise your SKU productivity
27 June 2022
2
min read