Top 10 Short-Term Replenishment Forecasting Challenges Retailers Face
Effective short-term replenishment forecasting is crucial in the complex and fast-paced retail landscape. It allows retailers to maintain optimal stock levels, minimize costs, and meet customer expectations.
However, retailers often need help with numerous challenges, so we asked Chat GBT to list the top ten short-term replenishment forecasting problems retailers frequently encounter. Based on this ranking and applying our experience working with major Retailers at Quantiful, we then offer some practical ideas on how to start solving them and how to evaluate any new AI tools you are considering as part of that solution.
1. Rapidly Changing Demand
Accurate forecasting is becoming more difficult as demand volatility continues to grow. Factors like seasonal trends, promotional activities, and sudden shifts in consumer behavior can cause rapid changes in demand, making accurate forecasting challenging.
Our advice: Start to consider tools that have two core capabilities. Firstly, automated alerting functionality can provide advanced notice of changes in demand in time for you to remediate them. Secondly, ensure the tools you are considering not only use your data for forecasting but also incorporate external data sets with advanced signals of those upcoming changes in demand patterns. The best tools use this data to improve forecast accuracy. More on this below.
2. Data Accuracy and Consistency
Traditional forecast algorithms rely heavily on historical sales data. Inaccuracies, inconsistencies, or gaps in this data can lead to incorrect forecasts, resulting in overstock or stock-out situations.
Our advice. Make sure any new tool you evaluate can automatically correct for historical data inconsistencies. For example, the best tools ensure an out-of-stock is not picked up by the forecasting algorithm as a lack of demand at that time, and a prediction of sales is automatically restored. This feature corrects the history and ensures a future forecast is consistent with actual demand.
3. SKU Location forecasting
Effective short-term replenishment becomes challenging where there is low demand, or particular SKUs have inherently low transaction activity across large SKU counts and complex distribution networks. Delays or inaccuracies can lead to misalignment between inventory levels and demand.
Our advice. It may seem obvious, but having tools that accurately build forecasts by SKU by Store is essential today. The trouble is if you have high SKU counts, a larger retail footprint or both, then most often, tools like Excel cannot handle the complexity and data loads. Again, ensure any alternative you are evaluating can provide that level of granularity in forecasting, not just at some clustered level like “all stores of the same size” but down to individual stores and their specific demand profile.
AI works by breaking a product down into its attributes and isolating which attributes most influence demand
4. Effect of Promotions
Promotional activities can significantly impact demand, often leading to sharp increases. However, accurately forecasting the impact of promotions can be difficult due to different promotional strategies' varying nature and effectiveness.
Our advice. The best consensus planning process combines product management intuition with the best AI forecasting tools, which illustrate the most influential drivers of demand in the past and future. Use specialised forecasting tools which use machine learning to understand the sensitivities of sales against your promotional activity. Then use these drivers of demand to scenario plan future promotional activity.
5. Supplier Reliability
Delays or inconsistencies in supplier deliveries can disrupt replenishment plans. Retailers must consider suppliers' reliability and lead times when forecasting short-term replenishment.
Our advice. The best AI tools deliver highly accurate forecasts over extended forecast horizons and have Administrator access-controlled UIs that can be shared securely with your vendor (or anyone who needs to see your forecast). This can be a read-only instance or an instance with controlled access provided to them to make forecast adjustments. These tools then allow comparisons of any variances they enter with your forecast, which can be surfaced in your consensus planning meetings.
6. Handling Product Variations
Products with many variants (like colour, size, and style) pose additional challenges. Each variant may have different demand patterns, requiring more granular forecasting.
Our advice. Much like the challenge introduced by complex distribution footprints, the best AI tools easily handle complexity at the portfolio level. As products have demand profiles, so do each product's attributes. Using machine learning, these tools can calculate size curves and colour and style variations to identify new product demand and an early indication of the end of life.
7. New Product Introductions
For new products without historical sales data, forecasting demand is particularly challenging. Retailers must estimate initial demand and subsequent sales patterns, often with limited information.
Our advice. This is where again, best-of-breed AI tools come into their own because of how they forecast. AI works by breaking a product down into its attributes and isolating which attributes most influence demand. So, if a new product shares any attributes with existing products, that will be used to generate a forecast. This means we can use predecessor identification on a many-to-many relationship of attributes. Further, if you use tools with external data, attributes from competitors or adjacent categories, to name two, it can be used to apply attributes extracted from new and emerging trends.
8. Cannibalization and Halo Effects
Sometimes, the sales of one product can impact another—this could be a cannibalization effect (where one product's sales decrease due to another) or a halo effect (where one product's sales increase due to another). Accounting for these effects in short-term replenishment forecasting can be complex.
Our advice. A significant limitation of emerging AI tools, even the largest, is their inability to forecast multiple time series simultaneously. Only the most advanced AI tools can calculate the relationship of demand across the whole portfolio. Ensure any tool you evaluate has this fundamental capability.
9. Returns and Damages
Returned or damaged goods can affect available inventory levels and must be factored into replenishment forecasts. However, predicting these rates accurately can be difficult.
Our advice. Inventory levels are treated like another data set and time series by the best AI tools and married to transactional sales. Variations from returns can then be accommodated to ensure forecasting considers “negative sales” in much the same way out-of-stocks underrepresent actual sales.
10. External Factors
External factors such as economic conditions, competitor activities, weather events, and more can influence demand and must be considered. However, the exact impact of these factors is often challenging to quantify.
Our advice. As mentioned under data challenges above, your new forecasting tool needs access to external data. It MUST also be able to forecast more than one time series of data, such as a critical external data set in addition to your transactional data time series, and integrate that forecast into a single forecast output. Even some of the biggest names in Software planning, like Microsoft D365, cannot do this. Make sure you ask and ensure any tool you evaluate can.
In conclusion, while short-term replenishment forecasting presents numerous challenges for retailers, it remains a crucial aspect of effective inventory management. By recognizing and addressing these challenges, retailers can improve their forecasting accuracy, optimize inventory levels, enhance customer satisfaction, and drive profitability. Emerging technologies like AI and machine learning offer promising solutions, helping retailers navigate these challenges with greater precision and agility.
QU from Quantiful has been designed to address many of these forecasting challenges, and if you would like to start an evaluation of QU with these requirements on your checklist, please get in touch.
Share this
You May Also Like
These Related Stories
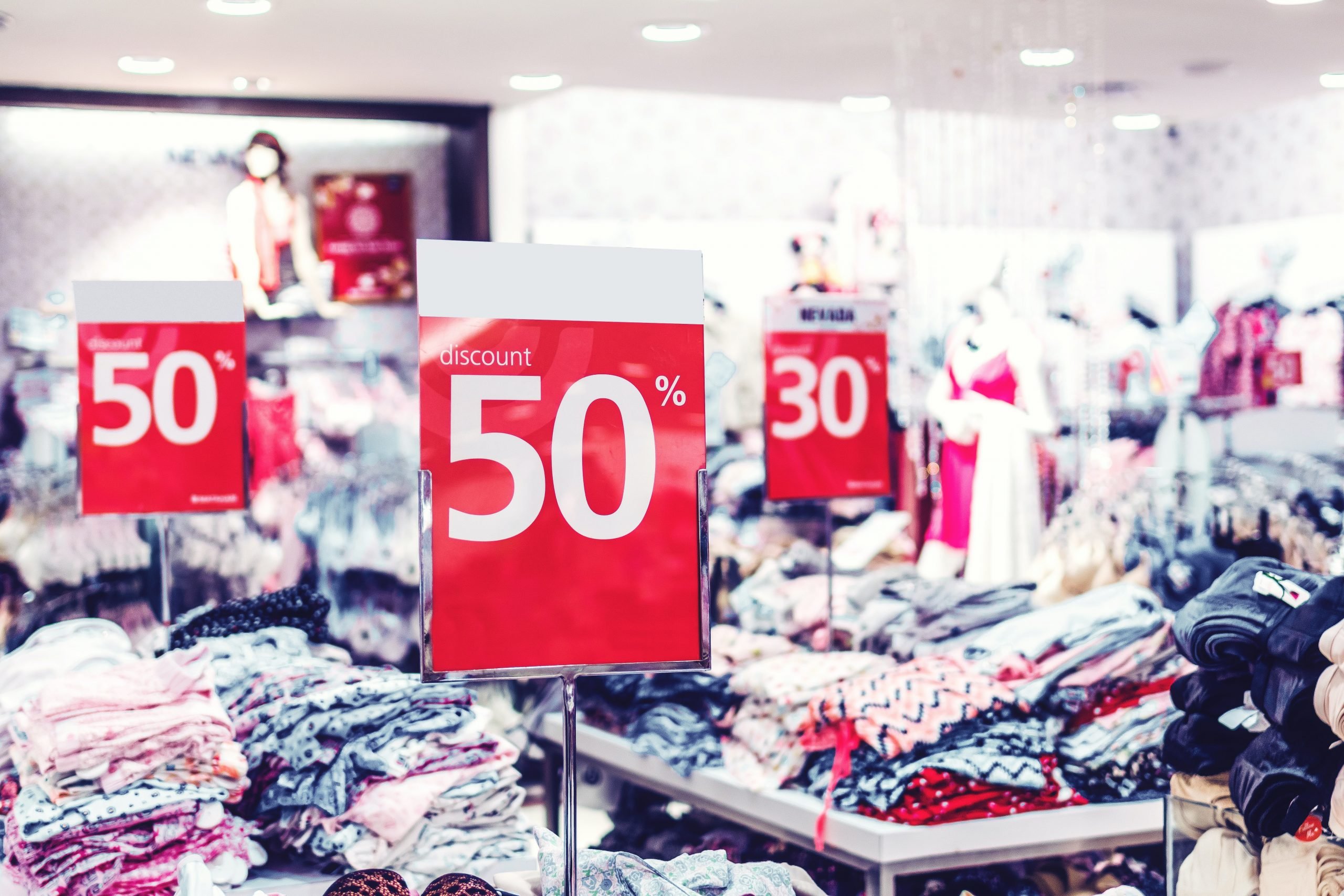
Optimise your replenishment process
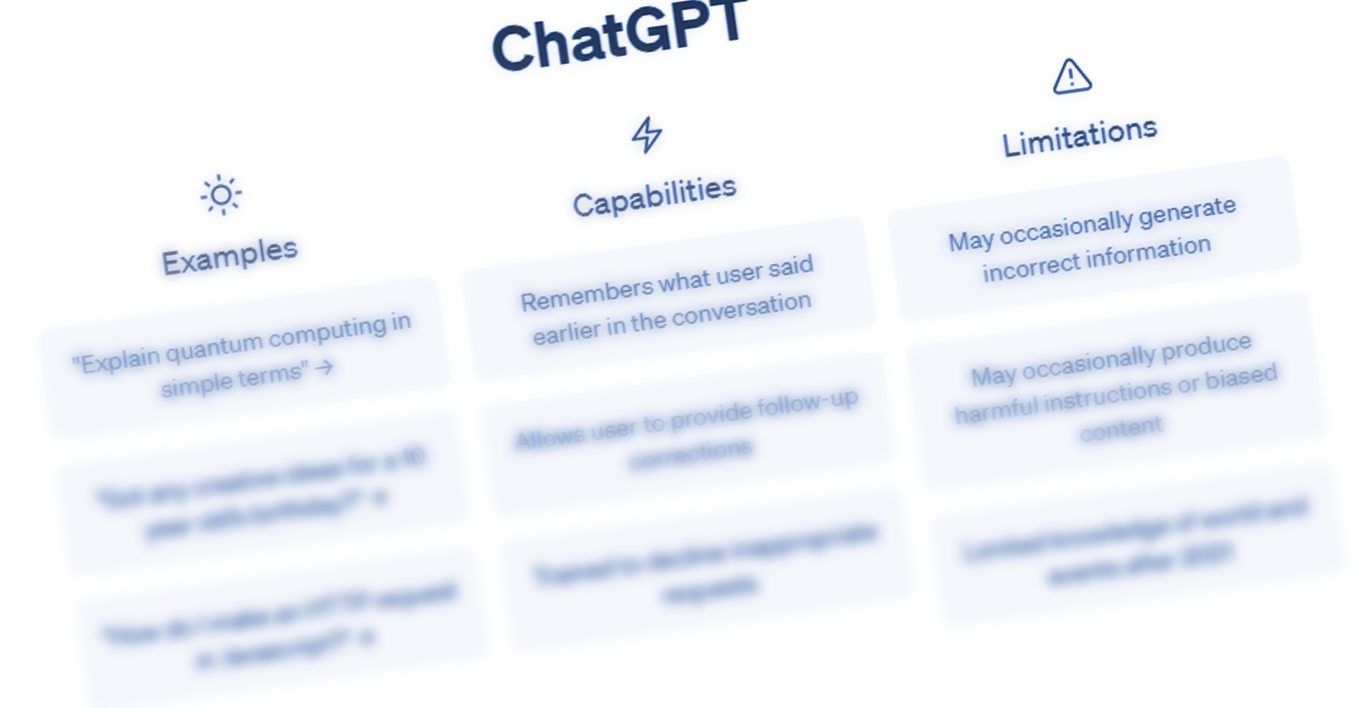
Will Generative AI tools like Chat GPT/GPT-4 replace Demand Forecasting tools?
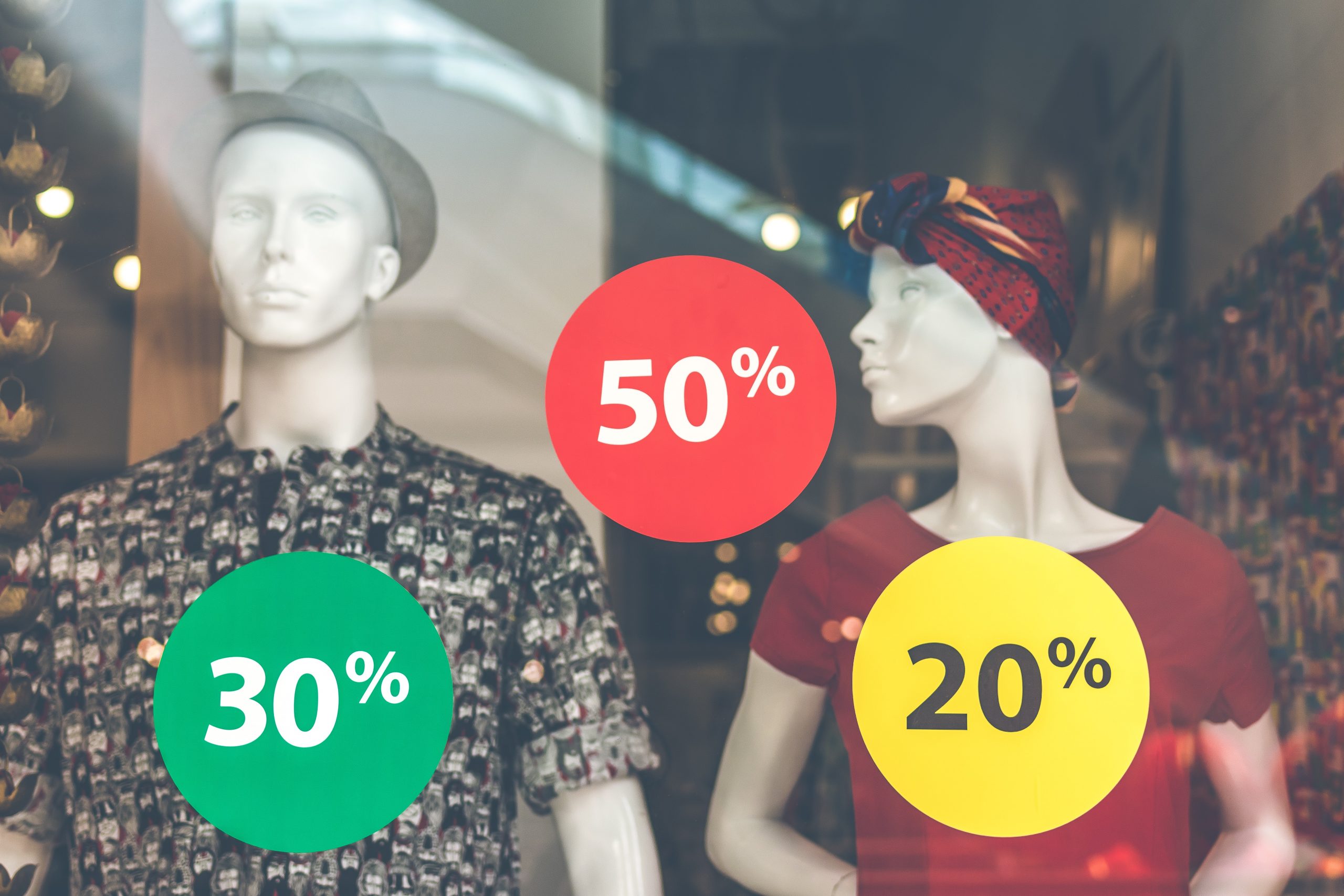