Forecast.
To predict or estimate a future event or trend.
Let’s start with a forecast we’re all very familiar with as an example - the weather. Once upon a time, forecasts were based on historical data captured around certain dates. Then, with the invention of telegraph networks, weather conditions could be observed and shared across larger geographic regions to predict changes. But these days, Meteorologists have the power to see what’s going on all over the world and with the help of computer modelling, can make ultra-precise predictions in real-time.
Demand Forecasting is no different. The science and technology in this space has moved on in leaps and bounds. Today’s tools have the power to give Demand Planners access to data-driven, actionable and explainable insight. These advancements are making the need to input reams of historical data into Excel all but redundant. Also drawing to a close are the days of formatting and maintaining epicly complex spreadsheets - which means more resource freed up to focus on strategy, and better continuity throughout staff changeovers.
Today’s tools have the power to give Demand Planners access to data-driven, actionable and explainable insight
Consider a new way to forecast demand
It’s been said that there are 6 types of Demand Forecasting:
- Passive Demand Forecasting
Using past sales data to predict the future - Active Demand Forecasting
Building in market research, marketing campaigns and expansion plans. - Short-term projections
Looking at just the next three to 12 months to manage your supply chain. - Long-term projections
Making projections one to four years into the future, taking into account historical data and future goals. - External macro forecasting
Incorporating trends in the broader economy and predicting how those trends will affect your goals. - Internal business forecasting
Factoring in your internal capacity to meet demand.
However, there’s a new methodology to add to the list that can do all of the above, and more.
AI, ML & Big Data-based forecasting
This next-generation method of predicting demand pulls on innovations in technology to enhance business operations and balance supply with demand. Not only can smart forecasting tools automatically pull in information from across your technology stack, they can scour carefully-curated data sets from all over the web and correlate findings with your business stats. This consolidation of internal and external trends, macro events, seemingly irrelevant anomalies and your business’s historical data is a powerful mix. Add the capability to constantly refine and learn and deliver real-time, actionable insights (thanks to AI / ML) and you have a Demand Forecasting tool that can reduce waste and seriously impact your bottom line.
All it needs is a skilled strategist at the helm.
Eyes front
Until recently, businesses spent a lot of time looking over their shoulders, with no choice but to use solely historical data in their demand predictions. The consequences were often dire. For example, in 1974 US Electric Utilities had planned to double their generation capacity by the mid-1980s. This was based on historical sales trends and a forecast of 7% annual growth in demand. The reality was that from 1975 to 1985, growth crawled along at just 2%, meaning the extra plants that were being built were not required. This is just one example of how relying solely on historical data can be a dangerous business.
Share this
You May Also Like
These Related Stories
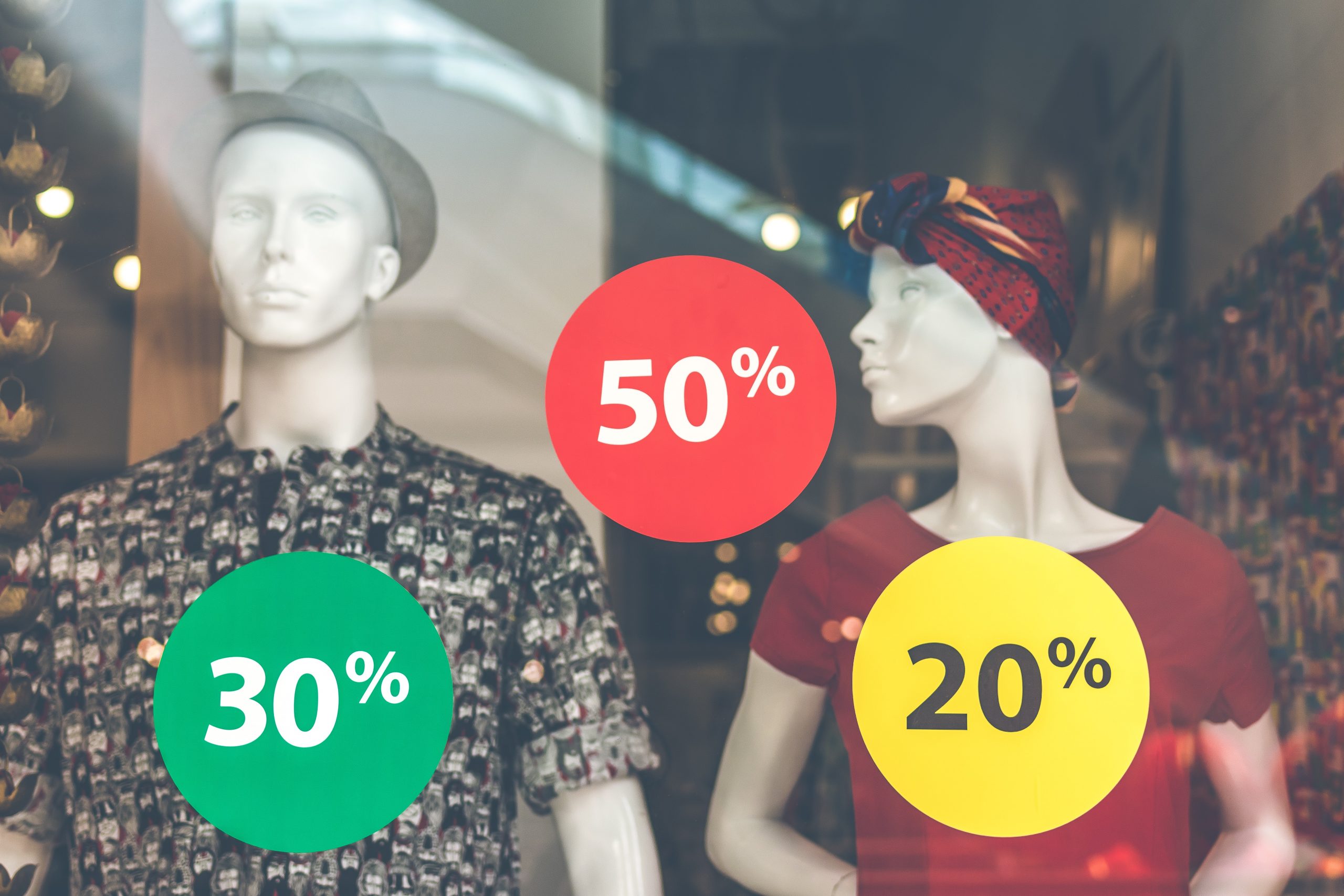
Move from demand forecasting to demand sensing
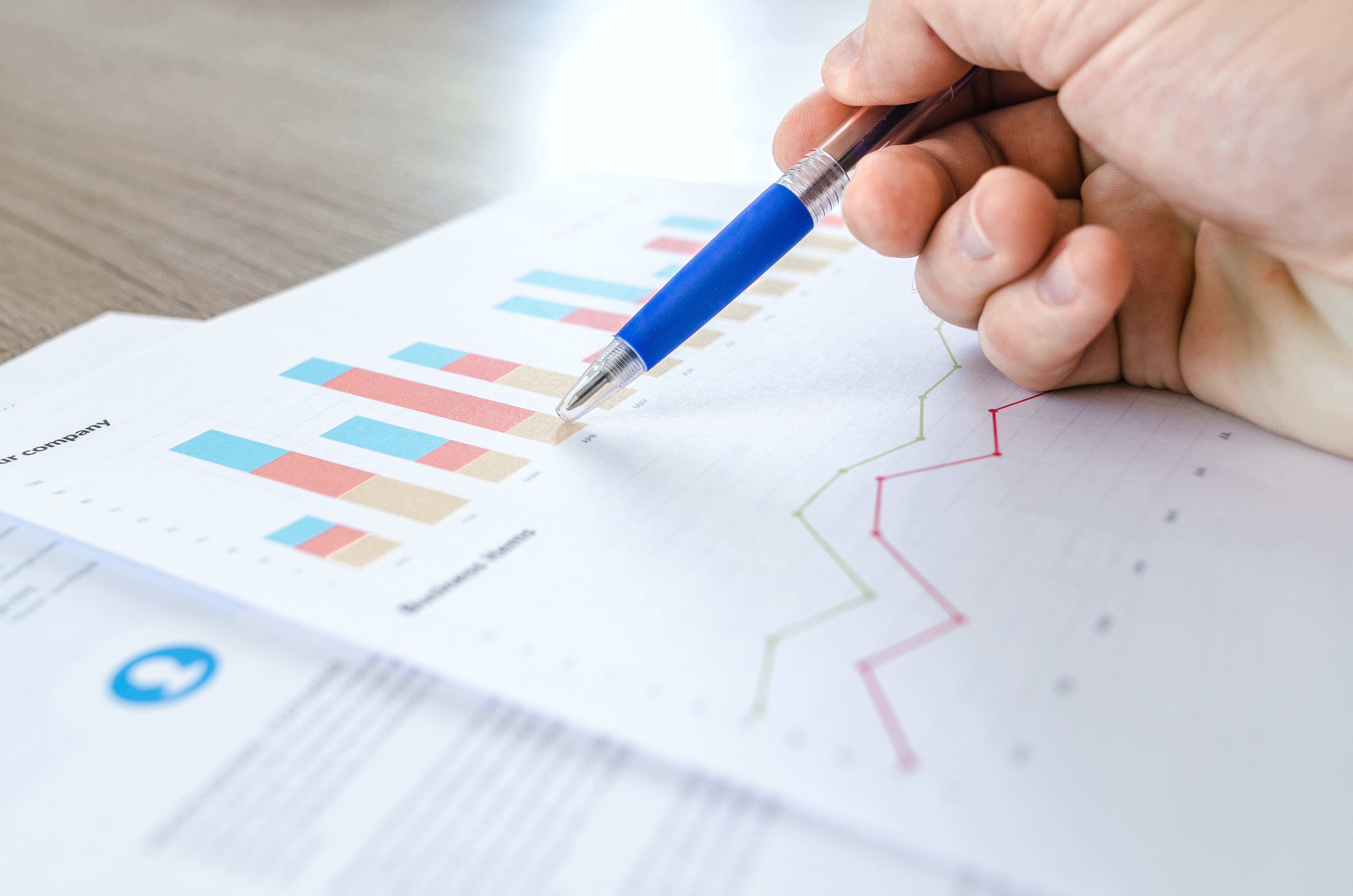
Top 10 Short-Term Replenishment Forecasting Challenges Retailers Face
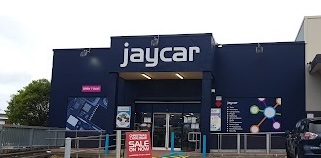