Calculating minimum stock levels in the age of AI
You already know that defining your safety stock level, minimum stock level or buffer stock is a key cornerstone of effective supply and demand planning. And no doubt you also understand all the formulas and processes that have been used for decades to set an “accurate” target that will ensure operational continuity and reduce the world’s waste levels.
But let’s be honest, no matter how flashy and complex your Excel sheet, using it to calculate safety stock is, quite literally, backwards. That’s because this process relies on retrospective data already generated by your business to set buffer levels and ensure seamless operation through market anomalies.
Today, there is a smarter way to define your minimum stock level. Recent innovation in AI (Artificial Intelligence) and ML (Machine Learning) means that businesses can now look ahead and predict changes in consumer behaviour, rather than simply reacting to them. These leaps forward in AI and ML, along with the enormous data sets that are generated by consumers every day, mean that businesses can more confidently make operational decisions that could make or break their retail business performance.
Flexible safety stock levels across complex businesses
People and process will always be vital when it comes to getting your minimum stock levels right. But a great team backed up with solid technology will always provide a more robust solution. When it comes to managing complex inventories across multiple locations, people can only ever do so much. But harnessing the power of the latest digital technology allows your business to fine-tune minimum stock levels across multiple locations, SKUs or regions. This ensures accuracy on a site-by-site or product-by-product basis, driving the overall accuracy of your minimum stock levels business-wide.
Ultimately, carrying less stock across your business is always going to free up capital to invest in potentially more profitable areas of your business, thereby maximising your overall performance.
Know what you need to, before you need to
Accurate forecasting that’s based on large and unbiased data sets helps you not just react to market anomalies, but predict them. With this information, you can get ahead of the curve - pivoting your product and distribution strategies to mitigate problems, before they arise.
With this information, you can get ahead of the curve - pivoting your product and distribution strategies to mitigate problems, before they arise
The limitations of traditional safety stock level processes
Previously, safety stock levels were based around key information that was generated by your business.
- How many units of an item are usually consumed within a given timeframe?
- What is the length of time associated with order processing and delivery?
Armed with this information, there were two formulas you could use to set your safety stock level. Both centred around usage and lead times that could only be worked out after a sales cycle.
The problem with this way of defining your safety stock levels is that the information required to optimise your demand planning can only be generated in the process of doing business. Basing your decisions soley on historical data is inherently flawed, as learnings will be made too slowly to implement effective product or distribution strategy changes. And because consumer behaviour is always changing, you can never be sure that your new-found learnings will apply to future market circumstances.
The problem with rigid minimum stock levels
When you are very rigid about your minimum (and maximum) stock level, you can end up accumulating more inventory than you need. It is therefore important that you continue to optimise your planning as you go and take a more flexible approach to stock levels. Working in this way will help you to reduce any expenditure associated with holding unnecessary stock, increasing your inventory turn and freeing up warehouse space for range expansion or other strategic business goals. Along with being inflexible, a manual process sucks up excessive people-power and so opting for an innovative system that can help you accurately forecast trends and adapt your demand planning will free up your people to focus on more fruitful endeavors, like research and development.
Why having AI and ML on your team is good for business
Whether you are a Demand Planner or a CEO, demand forecasts based upon vast swathes of data put you in a powerful position when it comes to effective decision-making. It’s not just about measuring and predicting buying trends against broad stroke indicators like consumer confidence or interest rates. Today’s leading organisations are using smart data capture to gain insight into consumer buying behaviour that is influencing the future of their sales. Everything from weather data, search data or data from sources that are leading indicators (e.g. building consents, mortgage rate increases etc.) can now provide vital forecasting for businesses.
If you would like to ditch the spreadsheet and find out more about optimising your replenishment planning, we have put together a helpful guide.
Share this
You May Also Like
These Related Stories
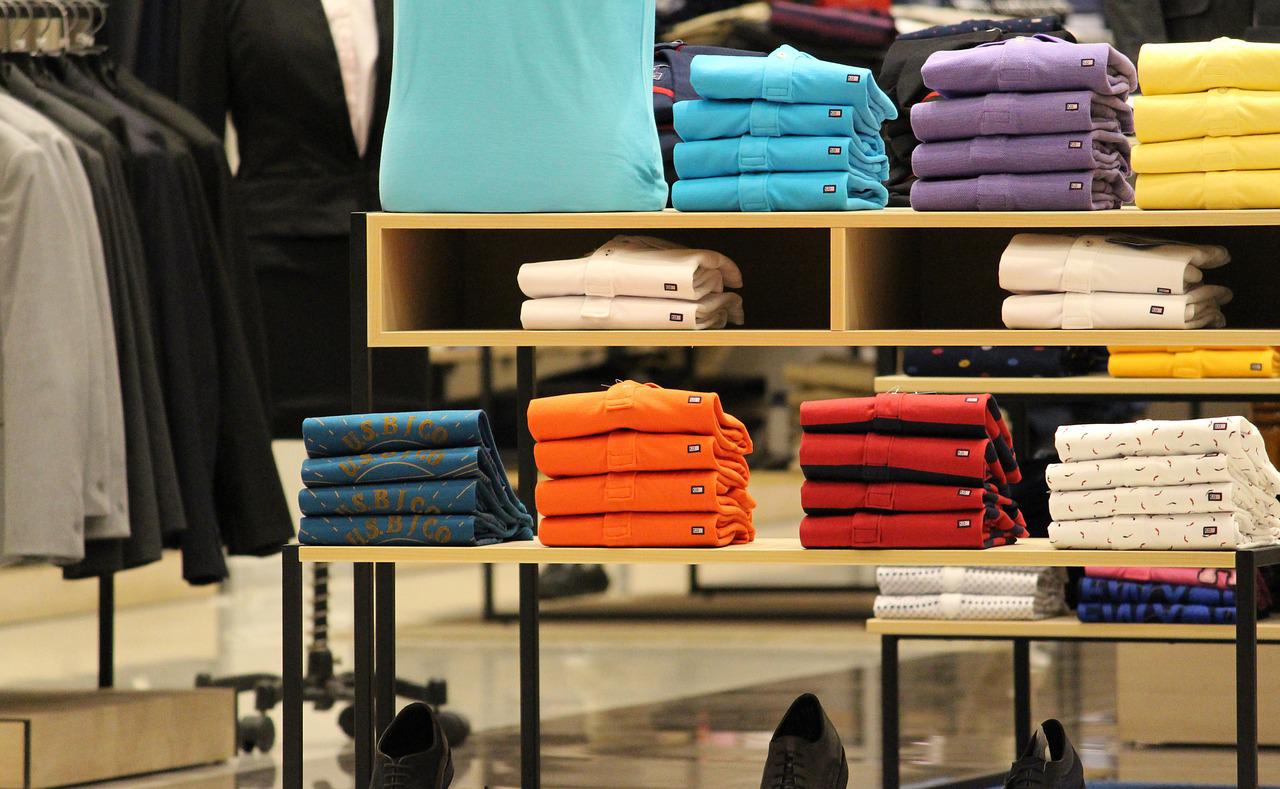
Transform your inventory management system
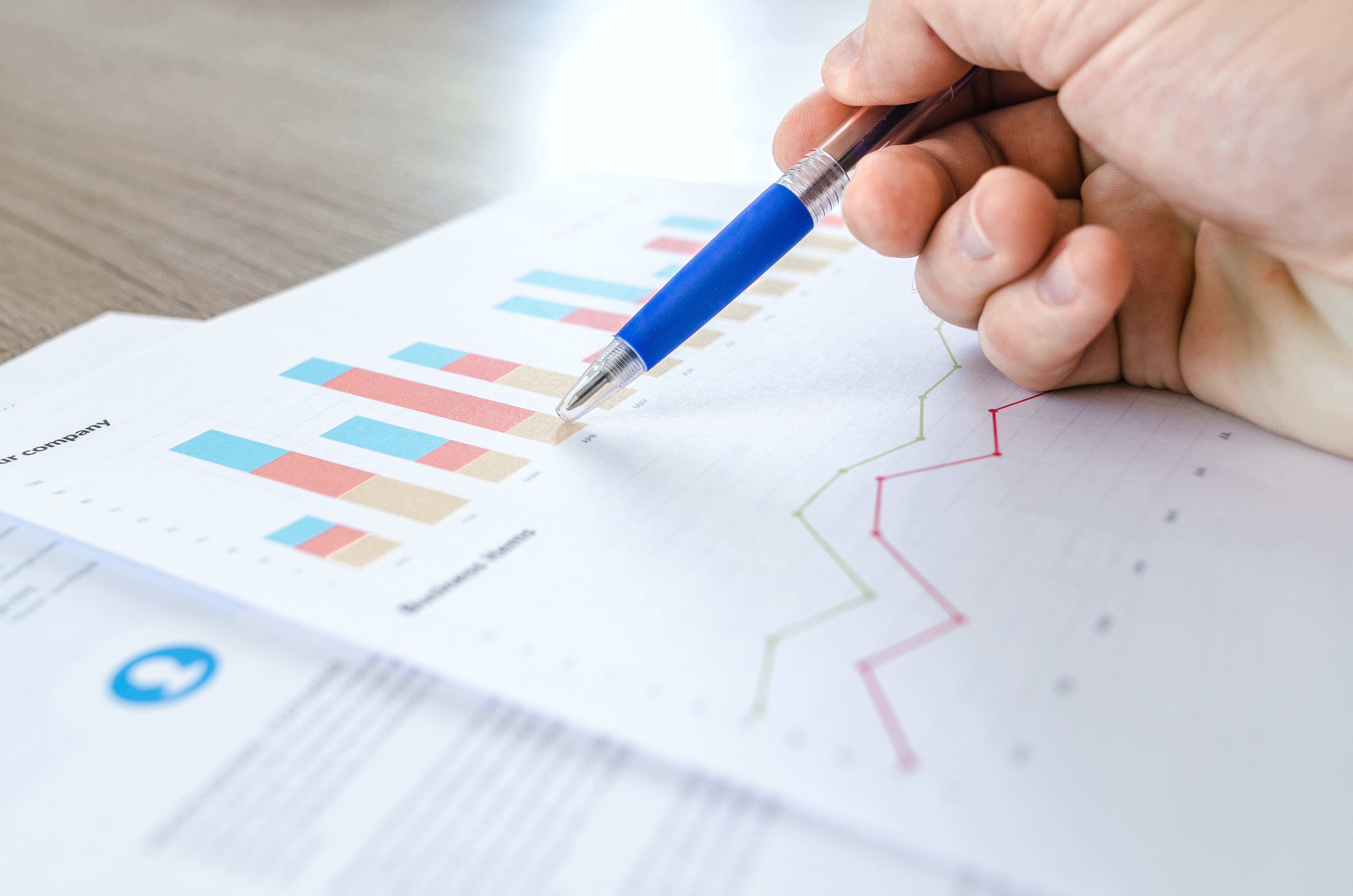
Top 10 Short-Term Replenishment Forecasting Challenges Retailers Face
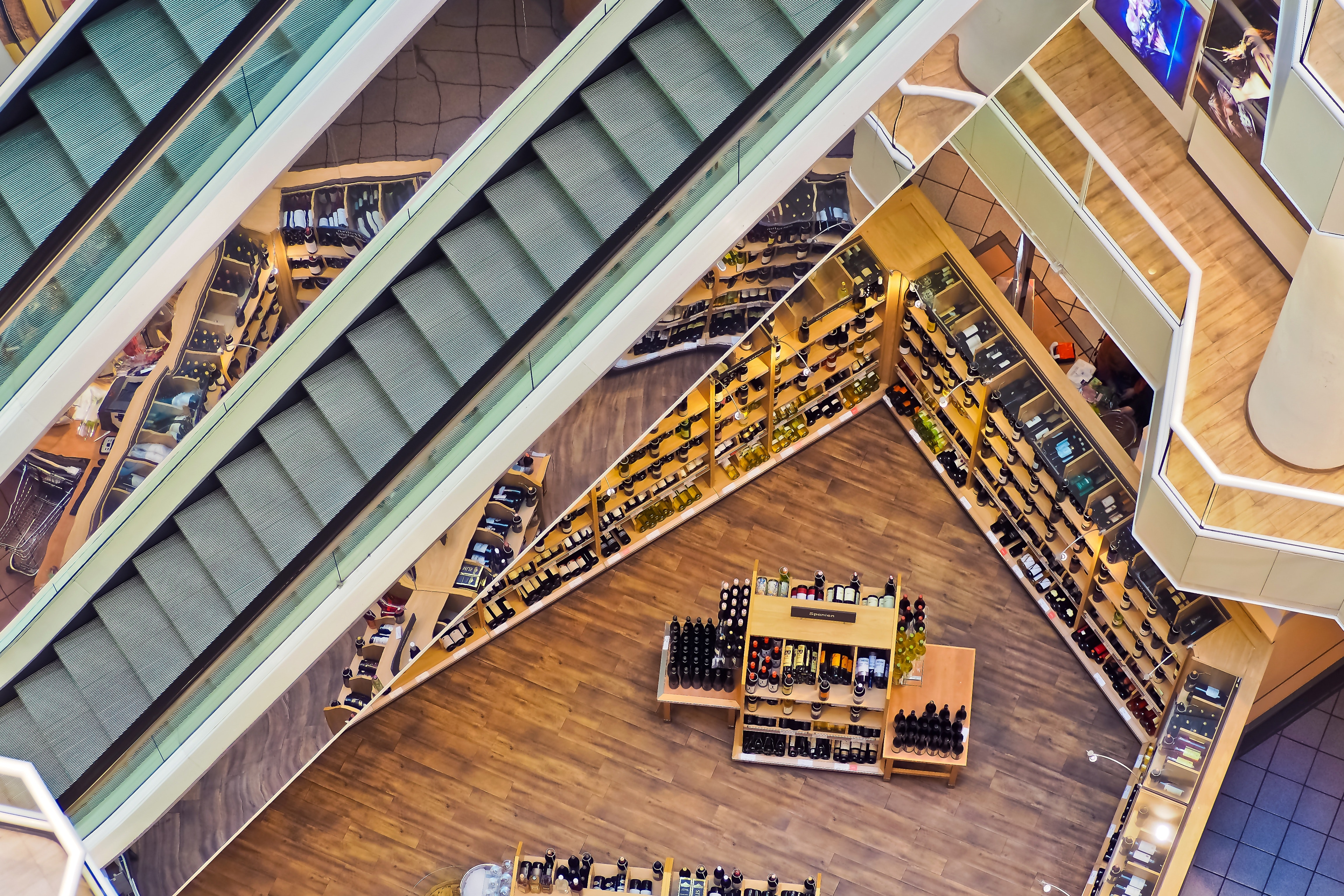